Monday, Nov. 18
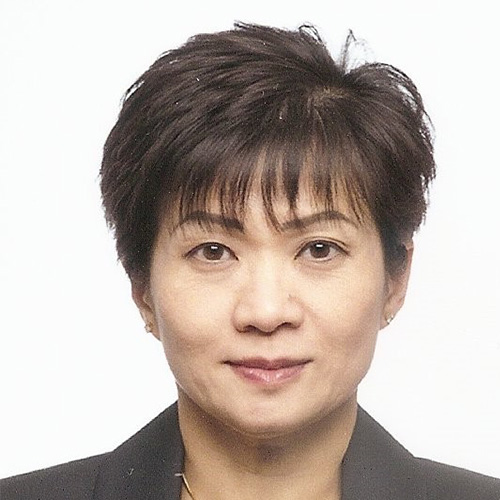
Thuc Hoang, National Nuclear Security Administration
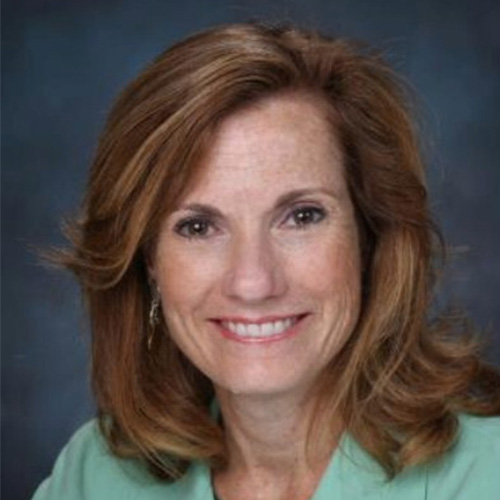
Trish Damkroger, Hewlett Packard Enterprise
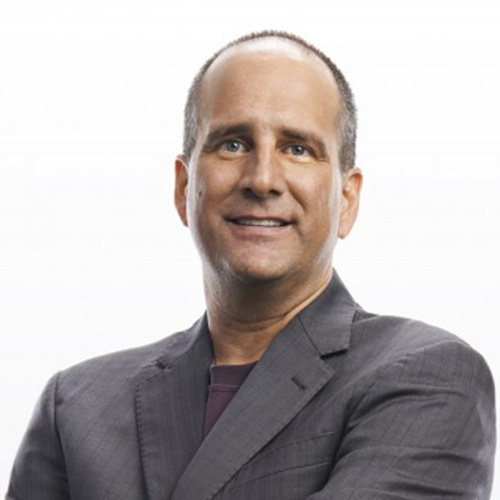
Brad McCredie, AMD
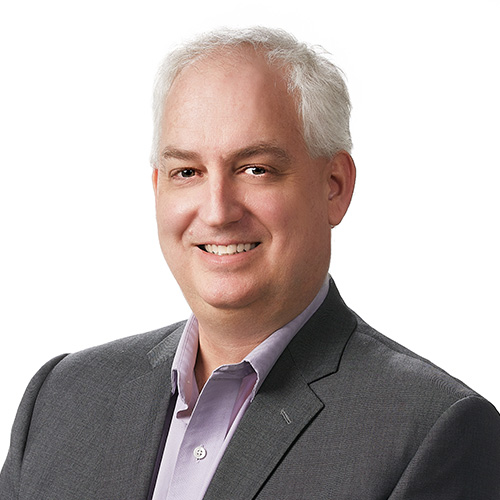
Rob Neely, Lawrence Livermore National Laboratory
Introducing El Capitan: Exascale Computing for National Security
7:45 P.M. EST
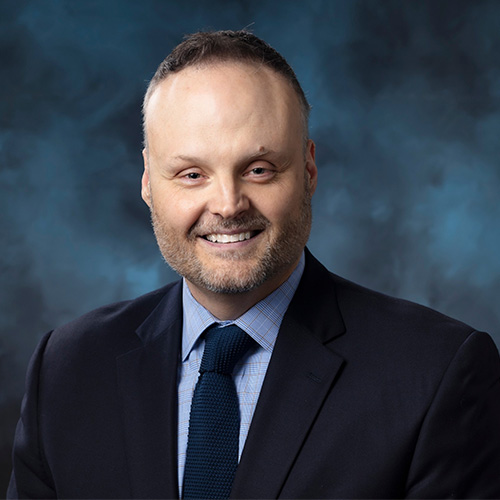
Bronson Messer, Oak Ridge National Laboratory
Fulfilling the Promise of the World’s First Exascale Supercomputer: Science on Frontier
8:30 P.M. EST
TUESDAY, NOV. 19
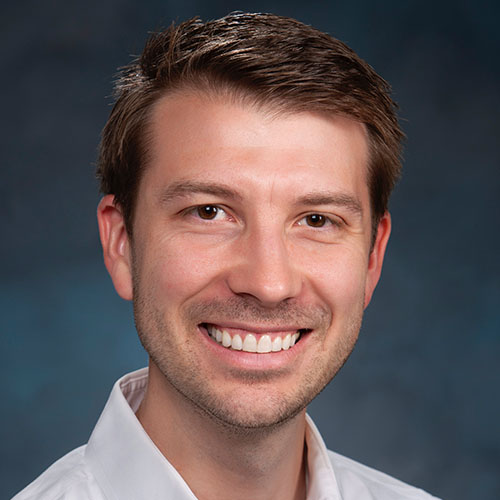
Luc Peterson, Lawrence Livermore National Laboratory
Harnessing Exascale: Revolutionizing Scientific Discovery with El Capitan
10:45 A.M. EST
» Abstract
Imagine wielding tens of thousands of accelerated processing units (APUs – combined CPUs and GPUs with unified memory) in an architecture designed for both scientific computing and artificial intelligence—what frontiers could you explore? El Capitan, the first exascale machine developed by the Department of Energy’s National Nuclear Security Administration (NNSA), stands on the brink of transforming our scientific and national security landscapes, boasting a staggering projected speed of over 2 exaflops.
In this discussion, we will explore the significant implications of exascale computing and its potential to drive breakthroughs in critical areas such as bioresilience, artificial intelligence, energy security, and stockpile stewardship. Central to this exploration is Lawrence Livermore National Laboratory’s Project ICECap (Inertial Confinement on El Capitan). Building on the landmark achievements of ignition and net fusion energy gain at the National Ignition Facility (NIF), ICECap seeks to harness artificial intelligence to enhance simulations and optimize designs, creating a prototype for the future of scientific discovery and paving the way for innovative solutions across various fields.
As we reflect on the collaborative journey that has led us here, we will consider how El Capitan embodies not just a leap in computational prowess but also a gateway to new realms of interdisciplinary collaboration and research. This transformative capability promises to unlock pathways to address some of the world’s most pressing challenges.
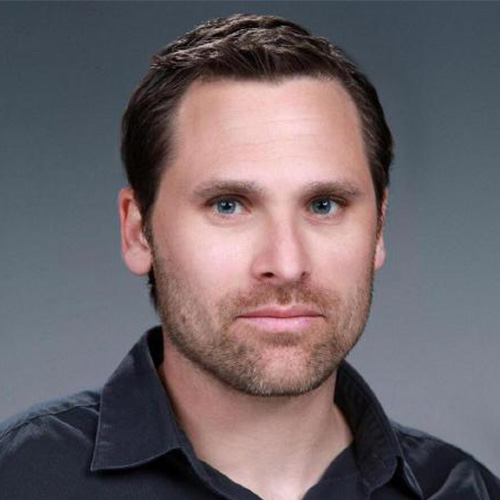
Malachi Schram, Jefferson Lab
Advancing Science Discovery using AI at Jefferson Lab
11:30 A.M. EST
» Abstract
In this presentation, we will discuss some new efforts in data science at Jefferson Lab focusing on enabling science discovery, improving operations at DOE Scientific User Facilities (SUFs), and helping study regional issues.
We will start by presenting a scalable asynchronous generative AI workflow designed for high-performance computing to solve fundamental nuclear physics questions which are at the heart of Jefferson Lab’s mission.
We will also explore how our research in AI/ML (e.g. conditional models, reinforcement learning, uncertainty quantification, etc.) can identify pending faults and improve operations at DOE SUFs.
Finally, we will present our research in AI/ML that addresses important regional issues (e.g. flooding and health informatics).
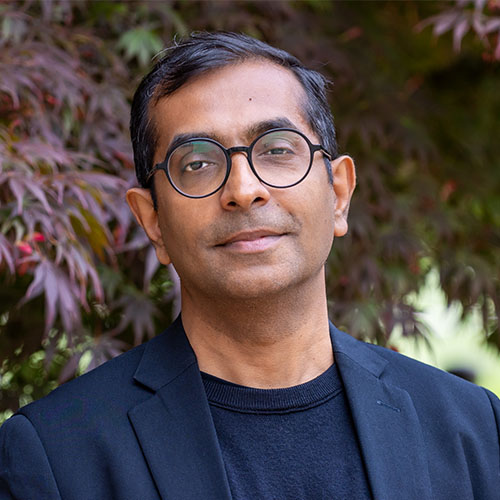
Shantenu Jha, Princeton Plasma Physics Laboratory
HPC is dead. Long live HPC: AI-coupled High-Performance Computing for Design and Discovery
1:00 P.M. EST
» Abstract
It is well known that traditional HPC approaches for simulation & modeling are rapidly reaching limits. We will discuss why and how AI-coupled HPC is necessary to overcome these limitations. We will present multiple examples of AI-coupled HPC workflow for scientific discovery and design by researchers at PPPL and Princeton in a broad range of discipline domains.
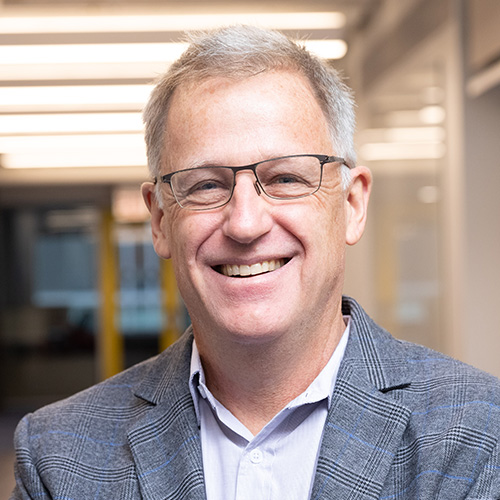
Ian Foster, Argonne National Laboratory
AuroraGPT: Scaling AI for Scientific Discovery
1:45 P.M. EST
» Abstract
Argonne National Laboratory’s AuroraGPT is an AI model designed to catalyze advancements in science and engineering that uses the Aurora exascale supercomputer. The primary goal is to build the infrastructure and expertise necessary to train, evaluate, and deploy large language models (LLMs) at scale for scientific research. Hear about what researchers have been doing to prepare the data used to train the model, efforts to evaluate the model, some early results, and how this project supports DOE’s Frontiers in Artificial Intelligence for Science, Security and Technology initiative.
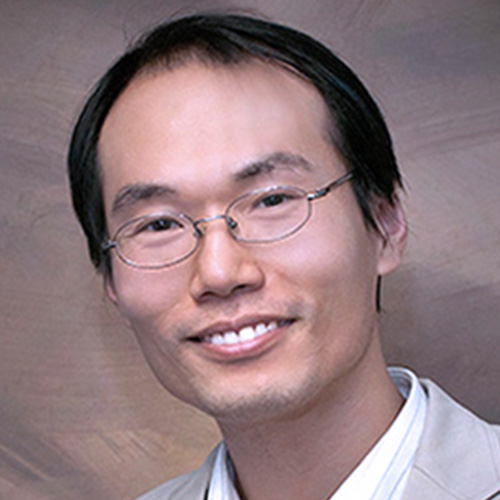
Shinjae Yoo, Brookhaven National Laboratory
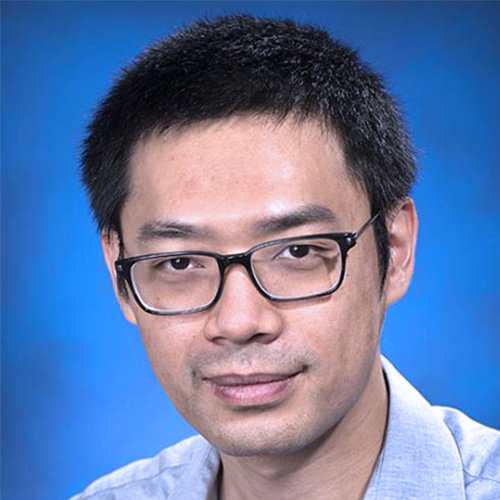
Yihui (Ray) Ren, Brookhaven National Laboratory
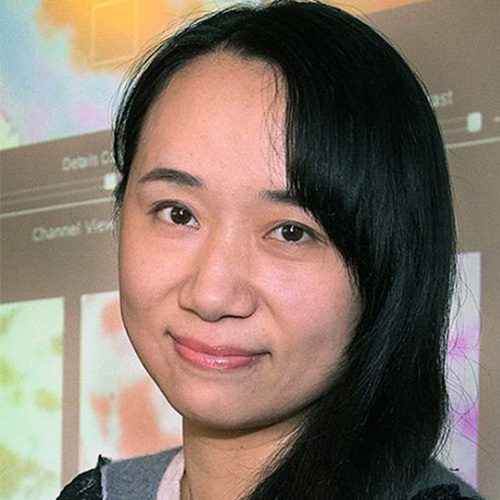
Wei (Celia) Xu, Brookhaven National Laboratory
AI for Science: From Infrastructure to Scientific Application
2:30 P.M. EST
» Abstract
The rapid evolution of artificial intelligence (AI) is transforming scientific research across domains. This talk, featuring Brookhaven Lab AI Department researchers, explores the role of AI in revolutionizing science with an emphasis on both infrastructure and application.
Initially, the focus will be on foundational models that enable large-scale scientific applications, underscoring the importance of building robust and secure AI systems. Then, the discussion will dive into the practical aspects of AI-driven solutions, such as UVCGAN, a generative adversarial network designed for unpaired image-to-image translation with U-Net discriminators, and BCAE, a bi-level autoencoder architecture for efficient data compression and transmission. Both models offer new approaches for handling complex data and enhancing scientific workflows. The talk also will cover optimization techniques for scientific workflows, including strategies for federated systems that balance data and workload distribution.
By addressing challenges from the hardware and infrastructure level to advanced applications, this talk will provide a comprehensive view of how AI can drive scientific discovery forward.
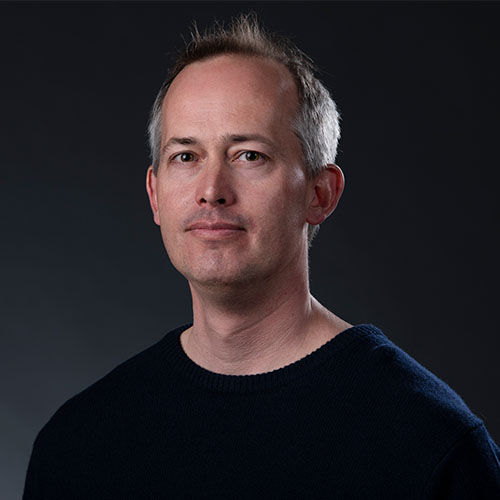
Earl Lawrence, Los Alamos National Laboratory
Artificial Intelligence for Mission
3:15 P.M. EST
» Abstract
The ArtIMis (Artificial Intelligence for Mission) Project is developing frontier AI to address mission challenges at Los Alamos National Laboratory. We are focused on two areas: (1) foundation models for science and (2) AI for design, discovery, and control. Methods are being developed and applied to four scientific domains: (a) materials performance and discovery, (b) biosecurity, (c) applied energy, and (d) multi-physics systems. In addition, we’re developing a cross-cutting capability in test, evaluation, and risk assessment for AI.
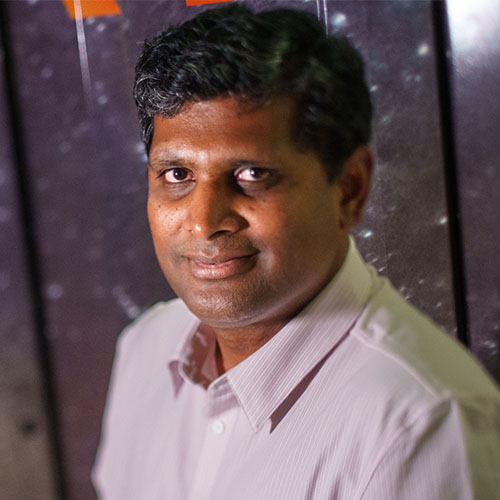
Siva Rajamanickam, Sandia National Laboratories
Turning the Titanic with a Leaf Blower: Co-designing programming models, libraries and advanced architectures
4:00 P.M. EST
» Abstract
In this talk, we will highlight some of our efforts for that past several years working with large and small hardware accelerator vendors in co-designing new architecture features, programming models, and software libraries. Our collaborations were at various levels from designing a backend in DOE performance portable software stack, new algorithms for linear algebra kernels, simulations to evaluate the usefulness of a new hardware feature for DOE applications. We will show examples from mini-applications, Gordon Bell finalists and production applications. This talk will also share some lessons learned for productive engagements in the future.
WEDNESDAY, NOV. 20
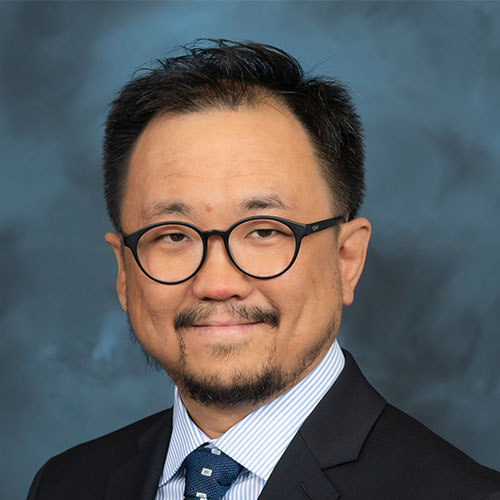
Woong Shin, Oak Ridge National Laboratory
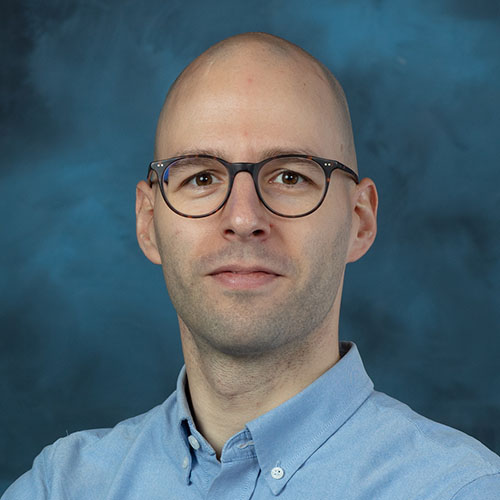
Matthias Maiterth, Oak Ridge National Laboratory
HPC Energy Efficiency @ Oak Ridge Leadership Computing Facility
10:45 A.M. EST
» Abstract
In this presentation, we will explore the OLCF’s journey from Summit to Frontier and beyond, focusing on enabling energy-efficient high-performance computing (HPC) for exascale achievements and the ongoing efforts towards the post-exascale era. The OLCF’s dedication to energy efficiency is highlighted by its power-efficient building infrastructure and system architecture, which are continuously improved through the utilization of operational data. This process involves the adoption of AI/ML models, visual analytics, and digital twins, progressively evolving into a data and software-driven energy-efficient user facility.
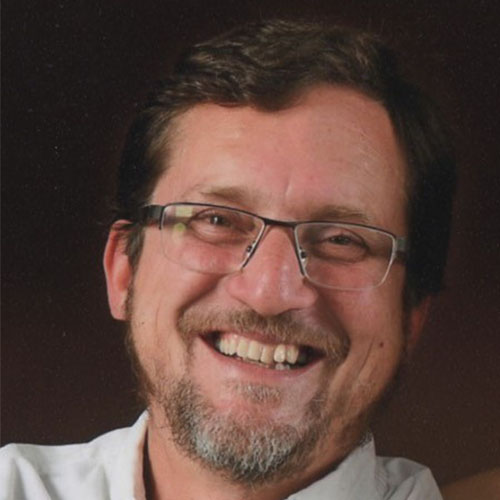
John Shalf, Lawrence Berkeley National Laboratory
Energy Efficient Computing
11:30 A.M. EST
» Abstract
John Shalf will discuss the rise of specialized architectures to boost HPC performance in the post-Moore’s Law era. Reminiscent of the so-called “Attack of the Killer Micros” heralding the arrival of microprocessors for HPC in the early 1990s, for the next generation of computing there is the potential for what could be called the “Attack of the Killer Chiplets” over the next five to 10 years to bring an era of HPC/AI modularity. This complements the emergence of similar in-memory or near-memory compute technologies such as Coarse-Grain Reconfigurable Arrays (CGRA), to enable specialized architectures, re-define computing and provide new avenues for advancing supercomputing speed and energy efficiency.
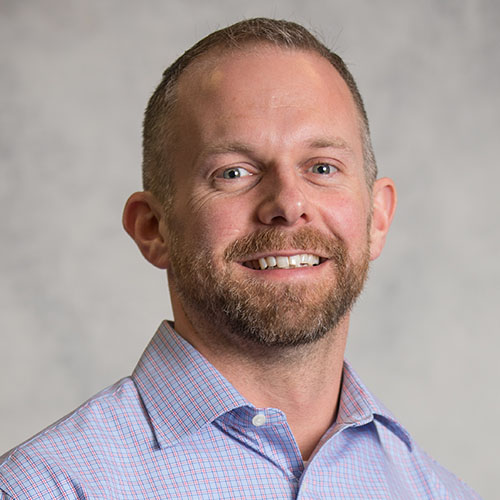
Jordan Musser, National Energy Technology Laboratory
Modeling and simulation of multiphase technologies
1:00 P.M. EST
» Abstract
This presentation provides an overview of the National Energy Technology Laboratory’s (NETL) multiphase computational fluid dynamics codes. The highly successful Multiphase Flows with Interphase eXchanges (MFIX) suite has been used to model a wide range of applications including post-combustion carbon capture, bioreactor optimization, and bio-FCC regeneration. MFIX-Exa, a state-of-the-art CFD code, developed under DOE’s Exascale Computing Project, is built on the AMReX software framework (https://amrex-codes.github.io/) and is designed to leverage modern accelerator-based compute architectures. This presentation further reviews the underlying physical models of both MFIX and MFIX-Exa and contrasts their similarities and differences. Examples of past and present CFD simulations will illustrate how scientific computing at NETL is being used not only for scientific exploration but also for design, optimization and scale-up of multiphase flow devices.
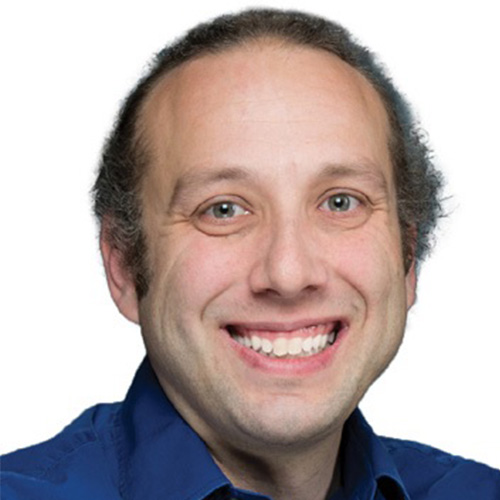
Aaron Fisher, Lawrence Livermore National Laboratory
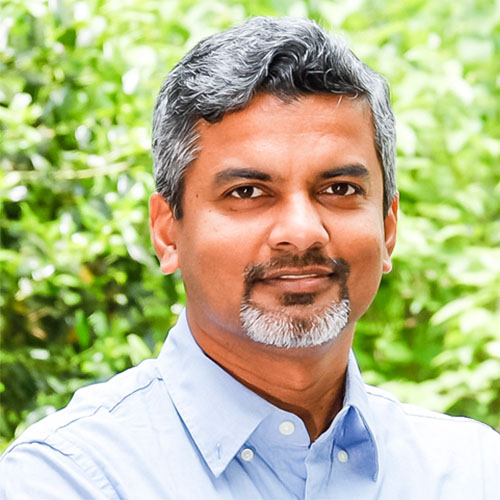
Ramanan Sankaran, Oak Ridge National Laboratory
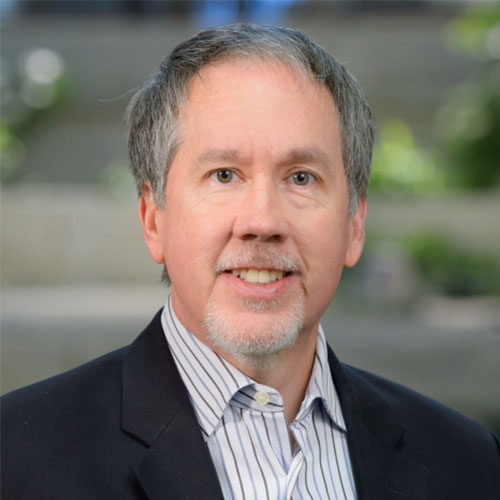
David Martin, Argonne National Laboratory
HPC4EI: Bringing national lab scale supercomputing to US Industry
1:45 P.M. EST
» Abstract
DOE’s High Performance Computing for Energy Innovation Program (HPC4EI) awards federal funding for public/private R&D projects aimed at solving key manufacturing challenges. Under the program, each selected industry partner gains access to the DOE National Labs’ supercomputers and expertise to help them solve large scale problems with the potential for signification energy and CO2 emission savings. These collaborative projects help these industries become more competitive, boost productivity, and support American manufacturing jobs.
Since 2015, HPC4EI has solicited, selected, and funded over 150 Cooperative Research and Development Agreements (CRADAs)—executed by national laboratory technology transfer offices—with over 50 U.S. companies including international brands such as Proctor & Gamble (P&G), General Electric (GE), Raytheon, General Motors, and Ford as well as startups refining their business concepts to seek capital for growth.
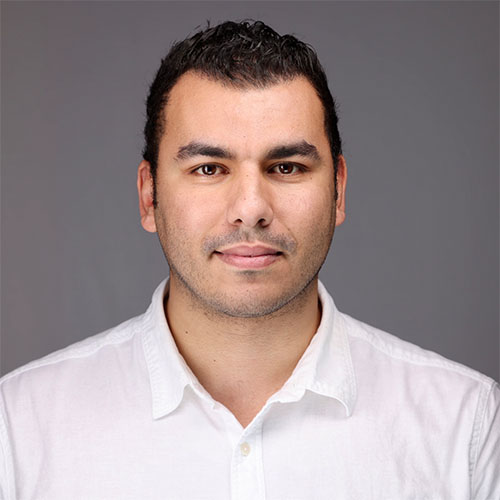
Walid Arsalane, National Renewable Energy Laboratory
HPC Multilevel User Environment Installation
2:30 P.M. EST
» Abstract
The National Renewable Energy Laboratory (NREL) has stood up the Lab’s third generation HPC platform Kestrel. Kestrel is a heterogeneous HPE/Cray system with 2,304 nodes with Intel Xeon Sapphire Rapids processors and 132 nodes with AMD Genoa processors. The AMD nodes contain 4 H100 GPUs. As with many HPC platforms the user environment is manifested via a module system. A number of factors contribute to the complexity of setting up a the user environment. These include the heterogeneous nature of the machine, newness of the architecture, the choice of module systems, the convolution of the base Cray module system, and having both OS and Cray provided development environments. There is also a desire to present users with options for building and running using more standard and/or newer software and compilers. We also provide access to a number of standard applications via the module system. This talk will discuss how we provided a useful environment on Kestrel. In particular we will discuss difficulties and how they were mitigated. The environment was set up using a combination of methodologies including, integrating with the base Cray system, appropriate set up of module initiation, builds from source, binary installs and builds/installs with spack. The spack builds include a multilayer buildout which aides in deployment but is mostly hidden from the user.
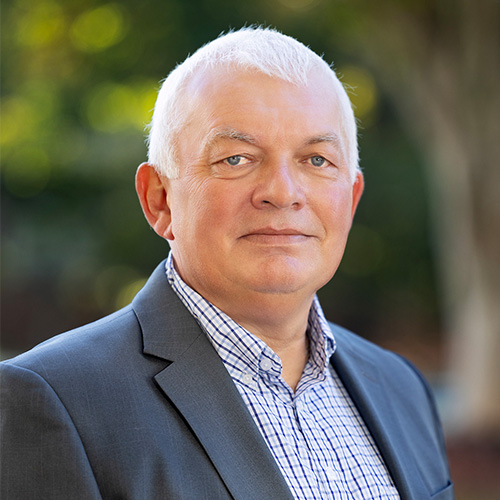
Graham Heyes, Jefferson Lab
High Performance Data Facility Status and Plans
3:15 P.M. EST
» Abstract
In October of 2023, the DOE awarded the lead of the High-Performance Data Facility project to the Thomas Jefferson National Accelerator Facility in partnership with Lawrence Berkeley National Laboratory. This presentation will discuss where we are today and our plans for the future of the project.
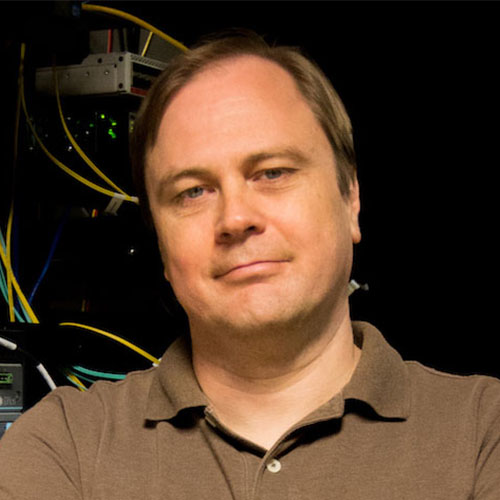
Ilya Baldin, Jefferson Laboratory
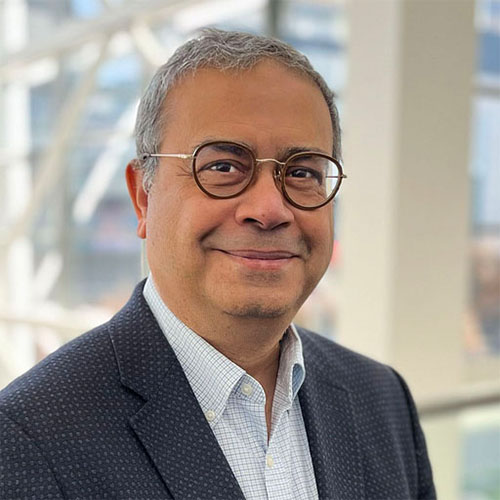
Yatish Kumar, ESnet
Implementing Time-Critical Streaming Science Patterns Using Distributed Computational Facilities
4:00 P.M. EST
» Abstract
This talk highlights the achievements, the current state and the future directions of EJFAT (ESnet JLab FPGA-Accelerated Transport) project in enabling real-time seamless streaming of scientific data at Terabit rates between instruments and widely distributed processing facilities. This real-time linking of the experimental and computational resources of the U.S. research enterprise is central to the Department of Energy concepts of IRI (Integrated Research Infrastructure) for the future of how science is done. It fully aligns with ESnet’s vision for the role the network should play in supporting cutting edge research and is critical to the design of the High-Performance Data Facility (HPDF) led by JLab. In this talk we will describe the custom hardware developed by ESnet, as well as the software framework developed by ESnet and JLab to build this technologically unique solution.
THURSDAY, NOV. 21
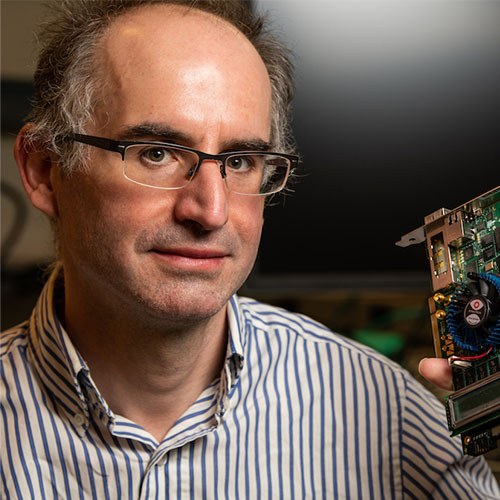
Antonino Tumeo, Pacific Northwest National Laboratory
Bridging Python to Silicon: the SODA toolchain
10:45 A.M. EST
» Abstract
Systems performing scientific computing, data analysis, and machine learning tasks have a growing demand for application-specific accelerators that can provide high computational performance while meeting strict size and power requirements. However, the algorithms and applications that need to be accelerated are evolving at a rate that is incompatible with manual design processes based on hardware description languages. Agile hardware design tools based on compiler techniques can address these limitations by quickly producing an application-specific integrated circuit (ASIC) accelerator starting from a high-level algorithmic description. In this talk I will present the software-defined accelerator (SODA) synthesizer, a modular and open-source hardware compiler that provides automated end-to-end synthesis from high-level software frameworks to ASIC implementation, relying on multilevel representations to progressively lower and optimize the input code. Our approach does not require the application developer to write any register-transfer level code, and it is able to reach up to 364 GFLOPS/W on typical convolutional neural network operators.
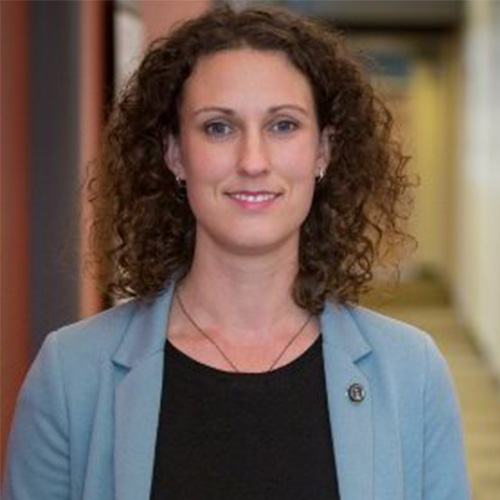
Debbie Bard, National Energy Research Scientific Computing Center
The DOE’s Integrated Research Infrastructure (IRI) Program Overview
11:30 A.M. EST
» Abstract
This presentation provides an overview of the Department of Energy’s Integrated Research Infrastructure (IRI) program, a comprehensive initiative designed to enhance and interconnect scientific research facilities across the nation. We will discuss how the IRI program aims to create a cohesive network of state-of-the-art laboratories, computational resources, and data sharing platforms. The talk will offer a brief overview of the program’s mission and goals, and Technical Subcommittees (TS) work, with particular emphasis on the TRUSTID TS.
» 2023 Featured Speakers
Monday, Nov 13.
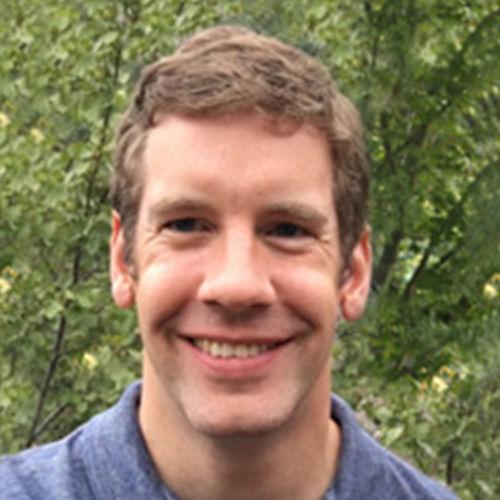
Todd Gamblin, Lawrence Livermore National Laboratory
“Introducing the High Performance Software Foundation (HPSF)”
» Abstract
The Linux Foundation is announcing the intent to form the High Performance Software Foundation (HPSF), which aims to build, promote, and advance a portable core software stack for high performance computing (HPC) by increasing adoption, lowering barriers to contribution, and supporting development efforts. Join us for remarks from the founding members and projects of HPSF!
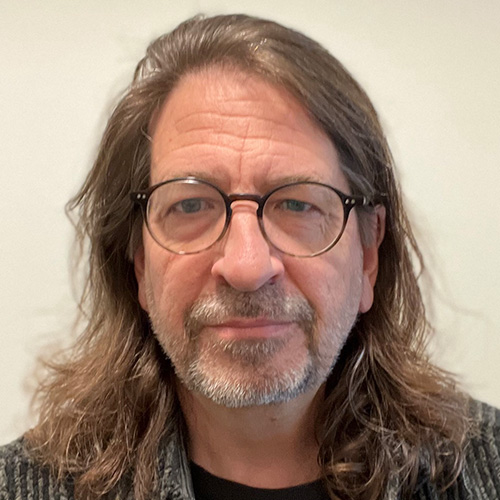
Rick Stevens, Argonne National Laboratory
“Introducing the Trillion Parameter Consortium”
» Abstract
A global consortium of scientists from federal laboratories, research institutes, academia, and industry has formed to address the challenges of building large-scale artificial intelligence (AI) systems and advancing trustworthy and reliable AI for scientific discovery. The Trillion Parameter Consortium (TPC) brings together teams of researchers engaged in creating large-scale generative AI models to address key challenges in advancing AI for science.
Tuesday, Nov 14.
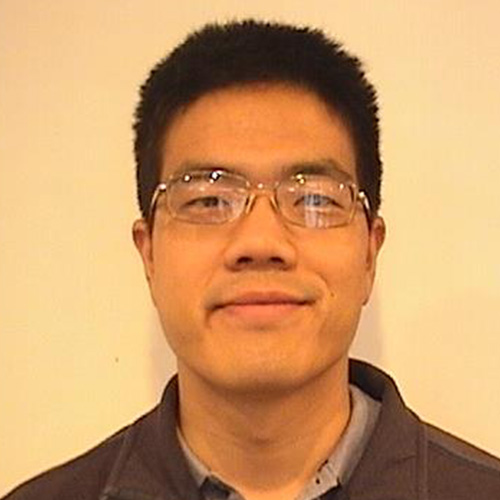
Stephen Lin, Sandia National Laboratories
“Performant simulations of laser-metal manufacturing processes using adaptive, interface-conformal meshing techniques”
» Abstract
Parts manufactured using laser-metal manufacturing techniques, such as laser welding and laser powder bed fusion additive manufacturing, are highly sensitive to the process details, which govern the formation of defects and distortions. Prediction of process outcomes requires models capable of representing both the complex dynamics present at the liquid metal-gas interface at the mesoscale and the overall macroscopic thermal response of the part. This work describes a model implemented in the Sierra mechanics code suite which uses mesh adaptivity and dynamic, interface conformal elements to capture both the high-fidelity physics at the mesoscale and the macroscopic thermal response of the part-scale. For the high-fidelity mesoscale model, the explicit representation of the interface in the dynamic mesh allows for simple and stable implementation of laser energy deposition, thermo-capillary convection, and vapor recoil pressure effects. For the part-scale macroscopic thermal response model, the build process for the entire geometry is resolved and restrictions on element sizes due to both the process layer height and laser spot size cause nearly intractable element counts without adaptivity. Improved adaptive meshing and load balancing strategies allow for scalable performance across thousands of cores and the efficient bridging of scales between laser and workpiece. Comparisons between our high-fidelity mesoscale model results and experimental data are presented as well as demonstrations of the model’s ability to predict pore formation, a key process-induced defect. Samples of the observed speed up and resource reduction for our part-scale models are also presented.
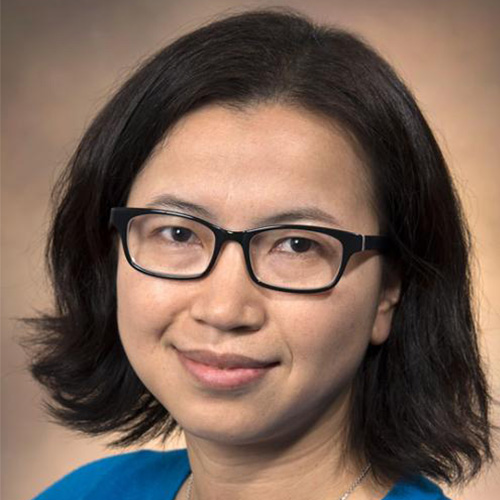
Meifeng Lin, Brookhaven National Laboratory
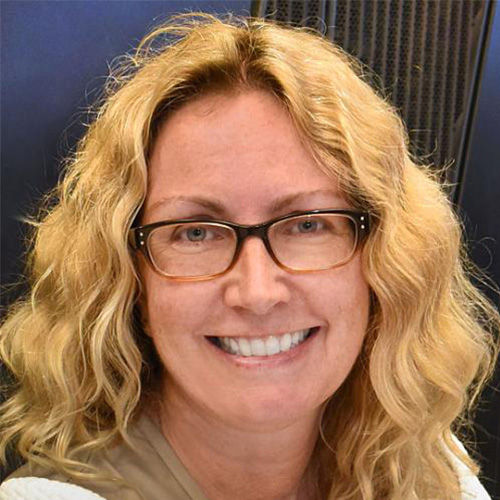
Kerstin Kleese van Dam, Brookhaven National Laboratory
“Digital Twins and the Rise of the Virtual Human”
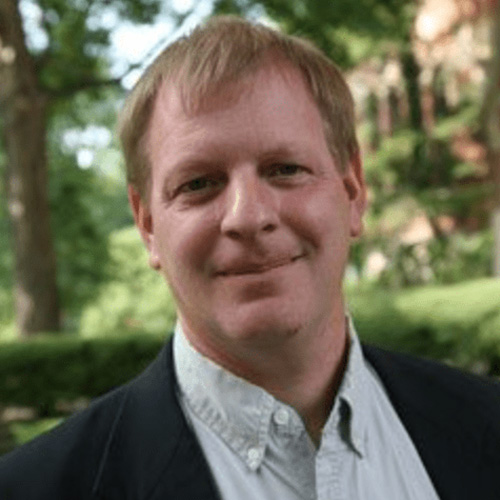
Eric Stahlberg, Frederick National Laboratory for Cancer Research
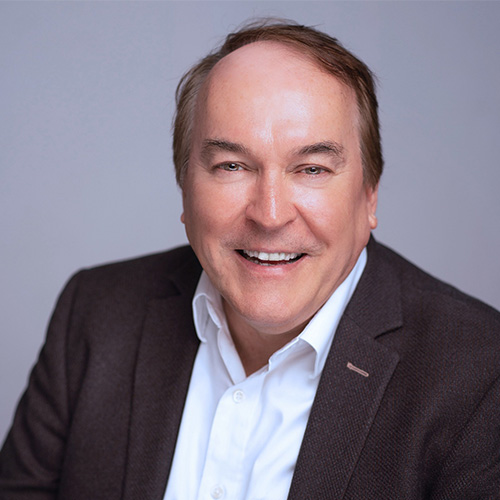
Peter Coveney, University College London
» Abstract
Biomedical Digital Twins, an innovative fusion of medical science and information technologies, are poised to transform medicine and improve patient care. These new approaches to medicine are just beginning to impact the fundamental understanding of biology and influence technologies available for preventing, detecting, diagnosing, and treating disease. Notably, the availability of Biomedical Digital Twins is opening exciting new avenues for exploring more personalized approaches to address specific health, wellness, and well-being needs of individual patients. This panel presentation will report on the outcomes of the recent Virtual Human Global Summit 2023 (https://www.bnl.gov/virtual-human-global-summit/), presenting an overview of state of the art in this domain, from academia and national labs to industry, and outlining future research directions.
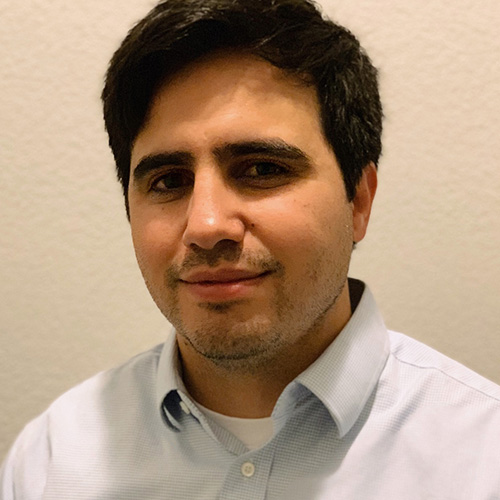
Dominic Manno, Los Alamos National Laboratory
“Leveraging Computational Storage for Simulation Science Storage System Design”
» Abstract
LANL has been engaged in how to exploit computation near data storage devices for simulation analysis. The activities in this area will be outlined with some early results and directions for the future will be covered.
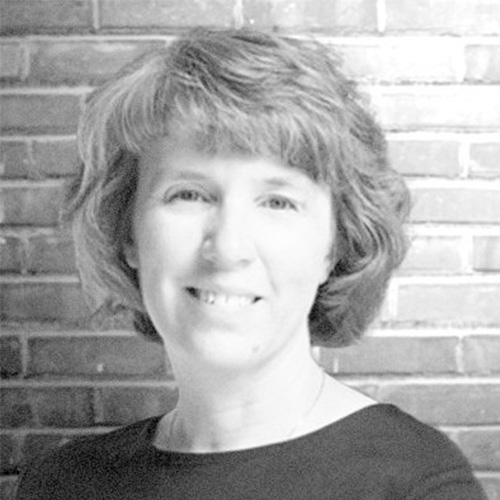
Lori Diachin, Lawrence Livermore National Laboratory
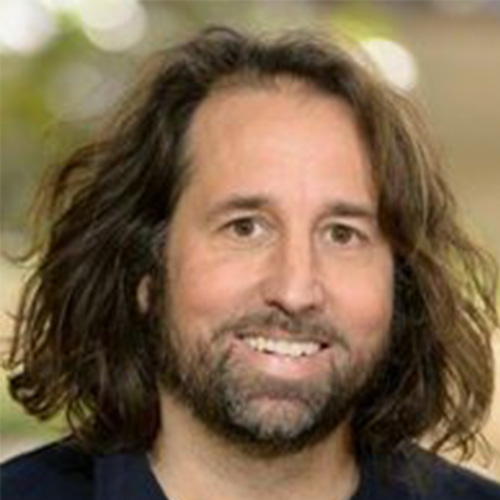
Andrew Siegel, Argonne National Laboratory
“Delivering a Capable Exascale Computing Ecosystem – for Exascale and Beyond”
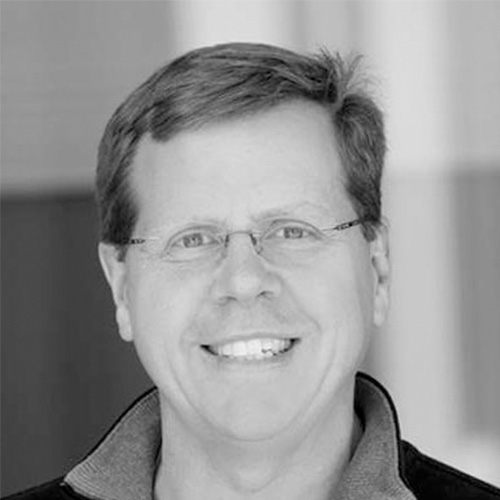
Michael Heroux, Sandia National Laboratories
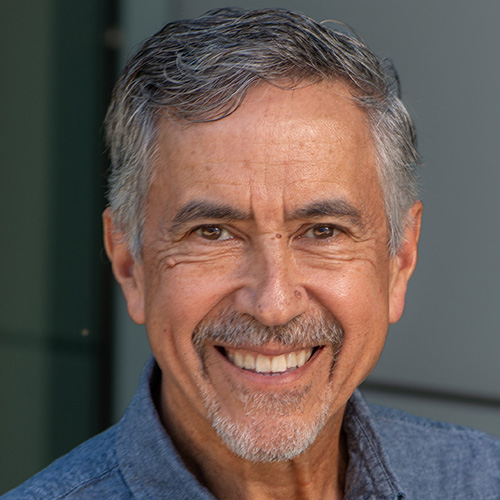
Richard Gerber, National Energy Research Scientific Computing Center
» Abstract
A close look at the massive co-design, collaborative effort to build the world’s first capable computing ecosystem for exascale and beyond. With the delivery of the U.S. Department of Energy’s (DOE’s) first exascale system, Frontier, in 2022, and the upcoming deployment of Aurora and El Capitan systems by next year, researchers will have the most sophisticated computational tools at their disposal to conduct groundbreaking research. Exascale machines, which can perform more than a quintillion operations per second, are 1,000 times faster and more powerful than their petascale predecessors, enabling simulations of complex physical phenomena in unprecedented detail to push the boundaries of scientific understanding well beyond its current limits. But the benefits of the exascale computing era will be far-reaching, impacting numerous aspects of HPC. This incredible feat of research, development, and deployment has been made possible through a national effort sponsored by two Department of Energy organizations, the Office of Science and the National Nuclear Security Administration, to maximize the benefits of high-performance computing (HPC) for strengthening U.S. economic competitiveness and national security.
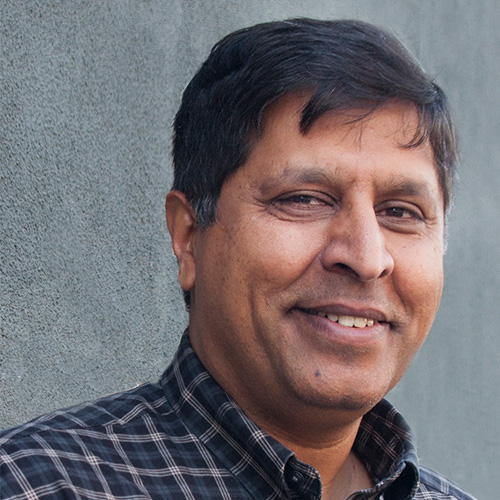
Sudip Dosanjh, Lawrence Berkeley National Laboratory
“The Next 50 Years: How NERSC is Evolving to Support the Changing Mission Space and Technology Landscape”
» Abstract
As NERSC heads into 2024 and its 50th anniversary, the center already has its sights set on the next 50 years. This is second nature for NERSC, which is always looking ahead to what is coming next in science, research, and technology as well as planning, installing, and refining its systems and services in response – and anticipation. Now, as we contemplate the future, NERSC is already evolving and expanding, with an eye toward both near- and long-term goals. These include: NERSC-10: Work on NERSC’s next supercomputer, NERSC-10, is underway, scheduled for arrival in 2026. The NERSC-10 system will accelerate end-to-end DOE Office of Science workflows and enable new modes of scientific discovery through the integration of experiment, data analysis, and simulation. AI and ML: HPC centers are preparing for a shift toward new AI-enhanced workflows, recognizing the need for future systems to leverage new technologies and support emerging needs in AI and experimental/observational science in order to accelerate workflows and enable novel scientific discovery. Preparation for NERSC-10 and future system planning are aligned with these imperatives. Superfacility: Since 2019, NERSC has led the Berkeley Lab Superfacility Project, formalizing the work of supporting this model and enabling better communication across teams working on related topics. In 2022, NERSC’s superfacility team participated in the DOE ASCR program’s Integrated Research Infrastructure Architecture Blueprint Activity, a project designed to lay the groundwork for a coordinated, integrative research ecosystem going forward. Quantum: Quantum information science and quantum computing hold promise for tackling complex computational problems. With the NERSC user base in mind, NERSC has been developing staff expertise and engaging scientists, QIS researchers, and quantum computing companies, initially through the QIS@Perlmutter program.
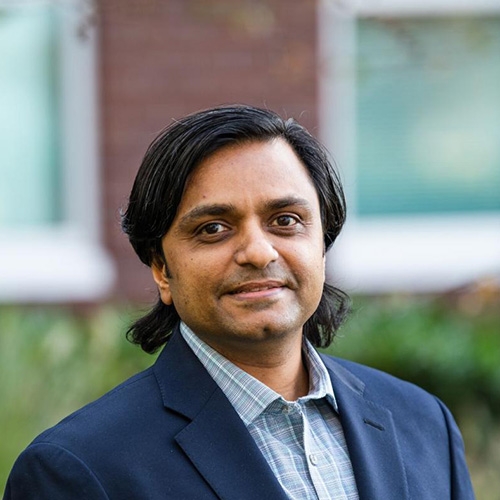
Mahantesh Halappanavar, Pacific Northwest National Laboratory
“ExaGraph: Graph and combinatorial methods for enabling exascale applications”
» Abstract
Combinatorial algorithms in general and graph algorithms in particular play a critical enabling role in numerous scientific applications. However, the irregular memory access nature of these algorithms makes them one of the hardest algorithmic kernels to implement on parallel systems. With tens of billions of hardware threads and deep memory hierarchies, the exascale computing systems in particular pose extreme challenges in scaling graph algorithms. The codesign center on combinatorial algorithms, ExaGraph, was established to design and develop methods and techniques for efficient implementation of key combinatorial (graph) algorithms chosen from a diverse set of exascale applications. Algebraic and combinatorial methods have a complementary role in the advancement of computational science and engineering, including playing an enabling role on each other. In this presentation, we survey the algorithmic and software development activities performed under the auspices of ExaGraph and detail experimental results from GPU-accelerated preexascale and exascale systems.
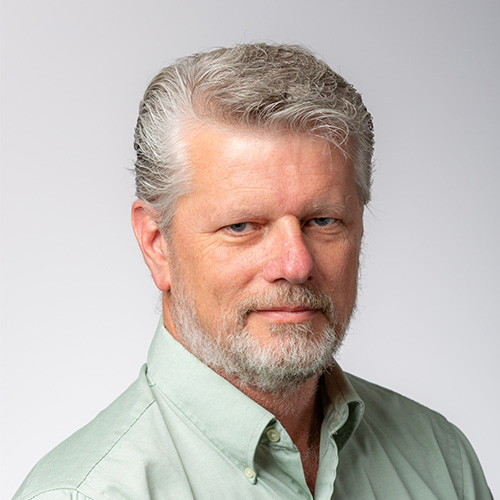
Marc Day, National Renewable Energy Laboratory
“Adaptive computing and multi-fidelity strategies for control, design and scale-up of renewable energy applications”
» Abstract
We describe our ongoing research in adaptive computing and multi-fidelity modeling strategies. Our goal is to use a combination of low- and high-fidelity simulation models to enable computationally efficient optimization and uncertainty quantification. We develop optimization formulations that take into account the compute resources currently available, which act as a constraint with regards to the fidelity level simulation we can run while maximizing information gain. These strategies are being implemented into a software framework with a generalized API allowing its application to a broad range of applications, from power grid stability and buildings control to material synthesis and biofuels processing. We will discuss a few examples from these applications that can benefit from this approach, especially when considering challenges arising in scaling up experiments and simulations.
Wednesday, Nov. 15
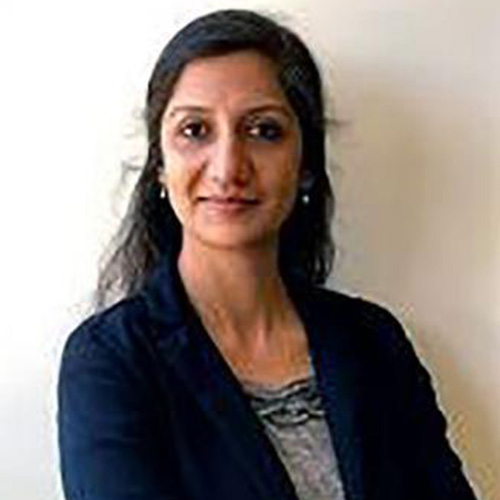
Rachana Ananthakrishnan, Argonne National Laboratory
“Nexus: Pioneering new approaches to integrating scientific facilities, supercomputing and data”
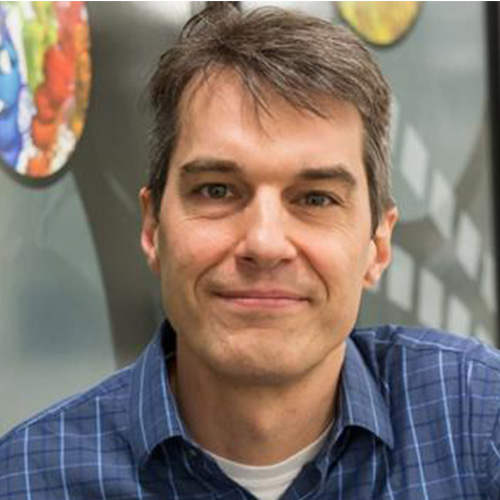
Tom Uram, Argonne National Laboratory
» Abstract
Nexus (anl.gov/nexus-connect), an Argonne initiative, connects experimental, computing, and storage facilities at Argonne and beyond into an interconnected scientific infrastructure. In so doing, it allows researchers to engage unique DOE resources at Argonne and beyond to tackle challenging questions, making the difficult easy, and the previously impossible conceivable. Building on the ubiquitous Globus research automation fabric, Nexus demonstrates what it means to achieve a lab-wide deployment of an integrated research infrastructure (IRI). Globus agents deployed on scores of computers, storage systems, and experimental facilities across Argonne provide for secure, reliable access to these varied resources. The resulting campus IRI enables innovative applications such as near-real-time analysis of experimental data, AI-directed control of self-driving laboratories, and interactive analyses of large datasets, both within Argonne and (thanks to Globus deployments at thousands of other institutions) across DOE and worldwide. IRI-focused innovations in computer and storage system configurations, including at the Argonne Leadership Computing Facility and Advanced Photon Source, support applications requiring on-demand computing and data integration. In this presentation, we will introduce the approaches the Nexus project has established for supporting integrated research infrastructure, and describe case studies of successful use across problem domains and facilities. The presentation will include a demonstration showcasing the user experience as a researcher defines and runs applications that span resources and facilities.
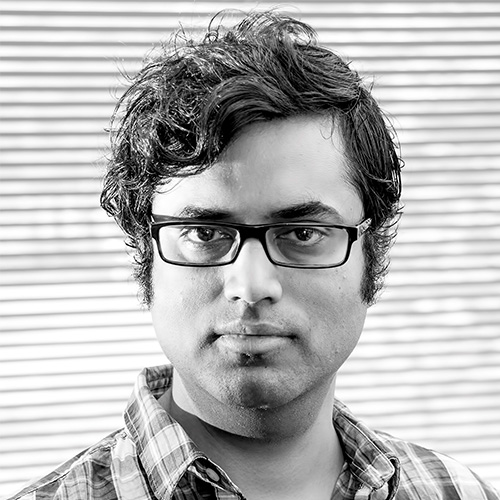
Ammar Hakim, Princeton Plasma Physics Laboratory
“Algorithms for Relativistic Kinetic and Fluid Simulations of Plasmas”
» Abstract
In this talk I will present recent innovations in the design of novel algorithms for relativistic simulations of plasmas. These span the regimes from fluids to kinetic continuum models, with applications ranging from laser-plasma interactions to the plasma environment around black holes. The algorithms I will show are of two sorts: finite-volume methods for plasma fluids, and discontinuous Galerkin methods for continuum kinetics. These schemes need to be designed with care as underlying physical properties, like total energy, entropy and kinetic energy must remain properly bounded. I will also describe the architecture of the software implementing these, and the progress made at PPPL in applying these models to various extreme relativistic physics problems.
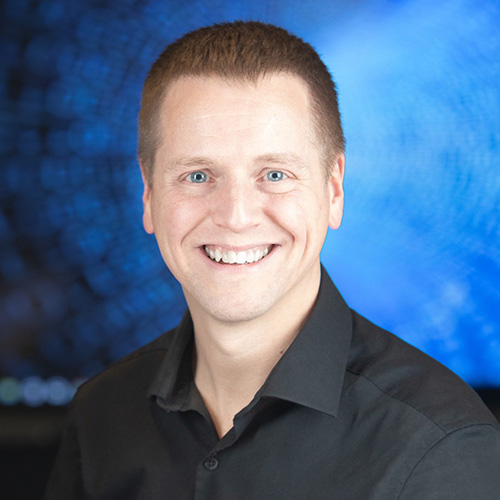
Trevor Wood, Oak Ridge National Laboratory
“Exascale use and impact on the future of flight”
» Abstract
To evaluate the potential efficiency delivered by an open fan architecture while simultaneously reducing noise levels, designers need to understand airflow behavior around the blades of the engine, including the complex physics of turbulence, mixing, and potential for flow for separation. GE engineers used Frontier to perform first-ever 3D Large Eddy Simulations at realistic flight-scale conditions with unprecedented detail toward revealing breakthrough insights into improving aerodynamic and acoustic performance in next-generation aircraft engine technology. The project lends crucial support to pursuit of reduction and eventual elimination of fossil fuel use for aircraft propulsion and achieve sustainable energy in flight. With its partner Safran, GE announced in 2021 the Revolutionary Innovation for Sustainable Engines (RISE) technology demonstration program to deliver over 20% lower fuel consumption and CO2 emissions compared to today’s most efficient aircraft engines. Fluid dynamics simulations on Frontier are enabling GE to extend learnings from prior research to realistic flight conditions and discover new ways of controlling turbulence, improve fan performance, and more efficiently guide future physical testing.
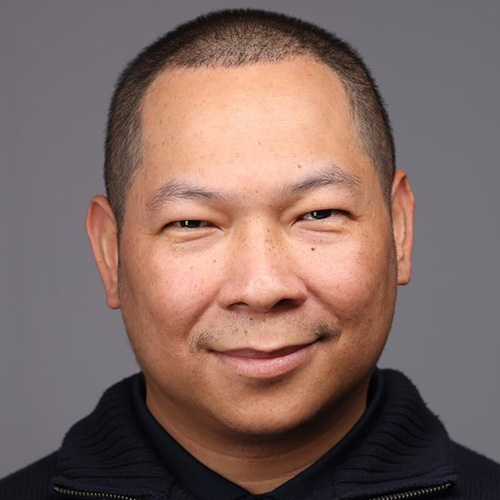
Sarom S. Leang, Ames Laboratory
“Enabling QM Study of Actual Length Scale Chemistry and Material Science”
» Abstract
The GAMESS (General Atomic and Molecular Electronic Structure System) software is a versatile computational chemistry package that facilitates understanding and predicting molecular structures and reactions from the quantum mechanical viewpoint. Leveraging a variety of computational methodologies such as Hartree-Fock (HF), Density Functional Theory (DFT), and various post-HF correlated methods, GAMESS provides researchers with an array of tools to investigate the electronic structure of molecules. The integration of accelerated methods into GAMESS represents a significant advancement, enhancing its computational efficiency and scalability. This session delves into the technical strides achieved in accelerating various compute kernels to electronic structure methodologies, including HF, resolution-of-the-identity second-order perturbation theory (RI-MP2), density functional theory (DFT), and resolution-of-the-identity coupled-cluster theory (RI-CC), onto modern GPU architectures.
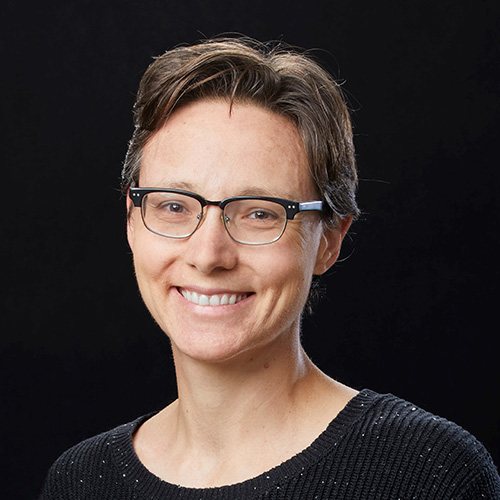
Jamie Bramwell, Lawrence Livermore National Laboratory
“High-Performance Multiphysics Applications at LLNL”
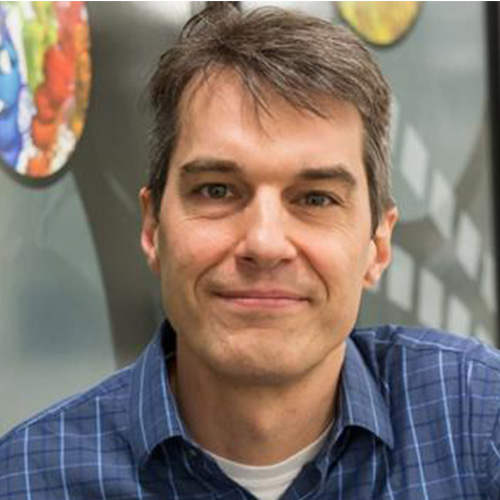
Aaron Skinner, Lawrence Livermore National Laboratory
» Abstract
High-performance multiphysics codes at LLNL support a variety of applications essential for predictive science at the NNSA. Our codes are large, complex, tailored to our applications, and represent decades of investment. Over the past 7 years, LLNL has invested heavily in single-source performance portability, and our codes are achieving breakthrough performance on GPU architectures. As part of our Multiphysics on Advanced Platforms Project (MAPP), we have also stood up a next-gen ICF, pulsed-power code, Marbl, using high-order methods based heavily on the Modular Finite Element Method (MFEM) library. As we prepare for the delivery of El Capitan, we have refactored our codes using a combination of performance portability abstractions and novel algorithm development. Our pursuit of computational performance at LLNL is not stopping, and as we look toward the post-exascale era, we believe the key to unlocking future performance gains will come from gradient-based optimization methods such as automatic differentiation (AD).
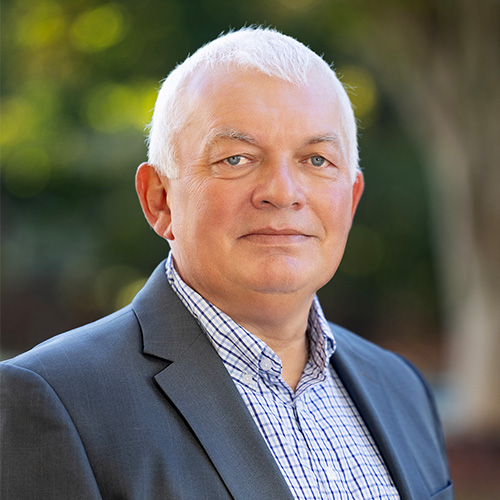
Graham Heyes, Jefferson Lab
“Research projects at JLab evaluating technologies for the High Performance Data Facility”
» Abstract
Several research and development projects are underway at JLab in partnership with other labs and ASCR facilities, particularly ESnet, to evaluate technologies and inform the conceptual design of the High Performance Data Facility (HPDF). This will be a new scientific user facility specializing in advanced infrastructure for data-intensive science. The Department of Energy recently announced that JLab will lead the project to build the HPDF Hub which will be developed in partnership with Lawrence Berkeley National Laboratory (LBNL), the ESnet host lab. The lead HPDF infrastructure will be hosted at JLab. This presentation will introduce the HPDF with focus on the technology demonstration projects and how they relate to the HPDF Hub project.
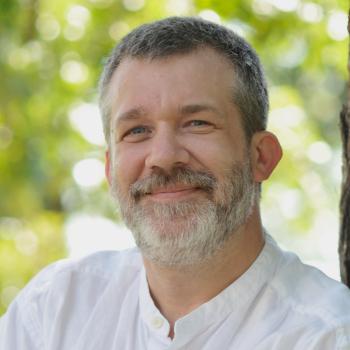
Charlie Catlett, Argonne National Laboratory
“Community Collaboration Around Large AI Models for Science”
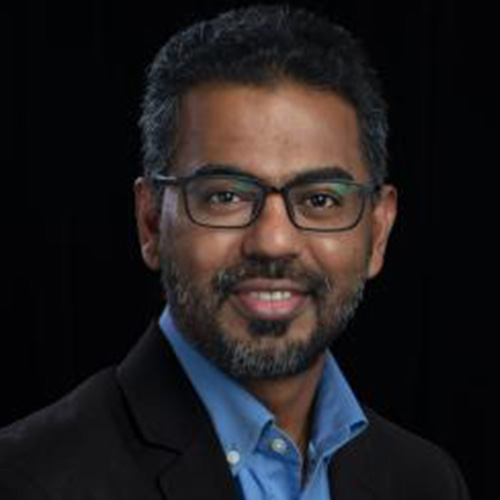
Prasanna Balaprakash, Oak Ridge National Laboratory
» Abstract
A new international effort is bringing together researchers interested in creating large-scale generative AI models for science and engineering problems with those who are building and operating large-scale computing systems. The goal is to create a global network of resources and expertise to facilitate the next generation of AI and maximize the impact of projects by avoiding duplication of effort.
Thursday, Nov. 16
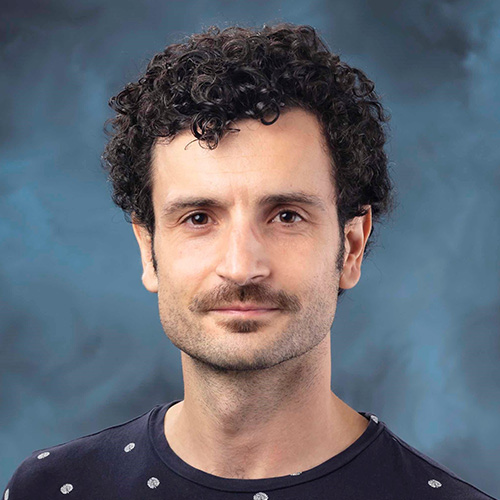
Damien Lebrun-Grandie, Oak Ridge National Laboratory
“Kokkos ecosystem – Sustaining performance portability at the exascale era”
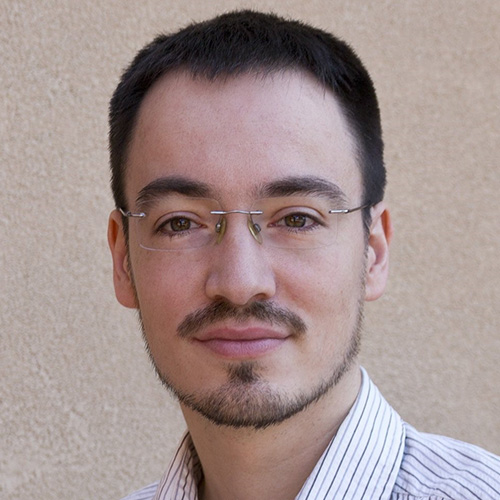
Christian Trott, Sandia National Laboratories
» Abstract
We have entered the exascale era of high-performance computing (HPC), and with it comes the challenge of writing software that can achieve high performance on a wide variety of heterogeneous architectures. The Kokkos Ecosystem is a performance portability solution which addresses that challenge through a single source C++ programming model that follows modern software engineering practices. Accompanied by a suite of libraries for fundamental computational algorithms as well as tools for profiling and debugging, Kokkos is a productive framework to write sustainable software. Today Kokkos arguably provides the leading non-vendor provided programming model for HPC exascale applications. Developed and maintained by a multi-institutional team of HPC experts, Kokkos is relied on by scientist and engineers from well over 100 institutions to help them leverage modern HPC systems. This talk will provide an overview of Kokkos and its benefits for performance portability and software sustainability. We will also present the Kokkos’ team vision for a community driven future of the project.
» 2022 Featured Speakers
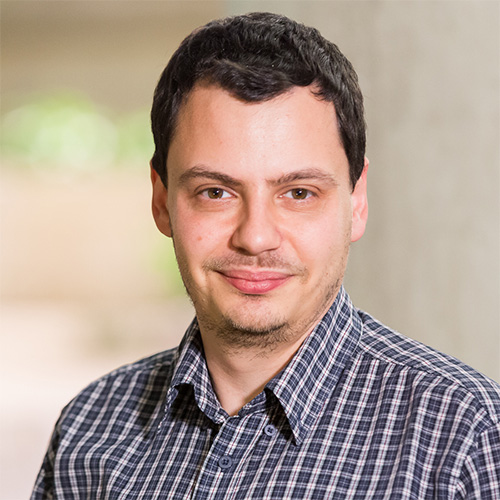
Bogdan Nicolae, Argonne National Laboratory
“Perspectives on the Versatility of a Searchable Lineage for Scalable HPC Data Management”
» Abstract
Checkpointing is the most widely used approach to provide resilience for HPC applications by enabling restart in case of failures. However, coupled with a searchable lineage that records the evolution of intermediate data and metadata during runtime, it can become a powerful technique in a wide range of scenarios at scale: verify and understand the results more thoroughly by sharing and analyzing intermediate results (which facilitates provenance, reproducibility, and explainability), new algorithms and ideas that reuse and revisit intermediate and historical data frequently (either fully or partially), manipulation of the application states (job pre-emption using suspend-resume, debugging), etc. This talk advocates a new data model and associated tools (DataStates, VELOC) that facilitate such scenarios. Avoid direct use of a data service to read and write datasets; instead, during runtime, users should tag datasets with properties that express hints, constraints, and persistency semantics. Doing so will automatically generate a searchable record of intermediate data checkpoints, or data states, optimized for I/O. Such an approach brings new capabilities and enables high performance, scalability, and FAIR-ness through a range of transparent optimizations. The talk will introduce DataStates and VELOC, will underline several vital technical details, and will conclude with several examples of where they were successfully applied.
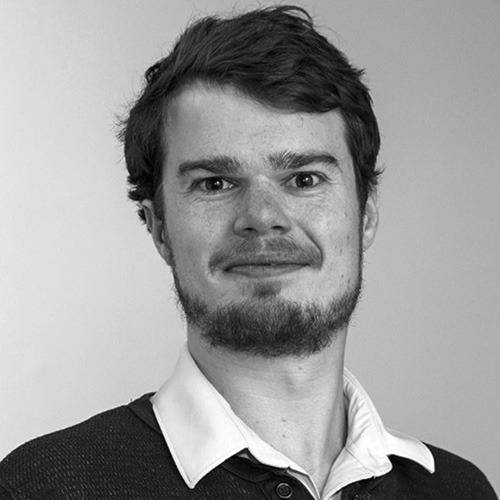
Andrew Tasman Powis, Princeton Plasma Physics Laboratory
“Beyond Fusion – Plasma Simulation for the Semiconductor Industry”
» Abstract
The Princeton Plasma Physics Laboratory (PPPL) has pursued and delivered excellence in scientific high-performance computing and algorithm design for many decades. This includes development of the gyrokinetic algorithm and delivery of code bases such as XGC, GTS, TRANSP, M3D and Gkeyll which are widely utilized within the burning plasma and heliophysics communities. Nonetheless, this foundation of skills in plasma physics, applied math and computer science readily lends itself to a more diverse set of applications and the laboratory is growing its efforts to facilitate computational modeling of low-temperature plasma (LTP) phenomena and plasma chemistry. LTPs are widely applied in industry, most notably within the semi-conductor manufacturing sector, which is predicted to double in value to over $1 trillion by the end of this decade. Combining its computational heritage and expertise in low-temperature plasma theory and experiments, the lab is developing a new open-source Low-Temperature Plasma Particle-in-Cell code to support this thrust. The software has been tested on NERSC’s Perlmutter and code validation is being performed in collaboration with experimentalists and industry partners around the globe. Additionally, we are leveraging codes such as LAMMPS and Gaussian to capture plasma surface chemical processes relevant to this domain of plasma physics. This talk will focus on PPPL’s legacy in high-performance computing and explain how the lab is leveraging that experience to tackle some of the greatest challenges facing our world today using advanced supercomputers.
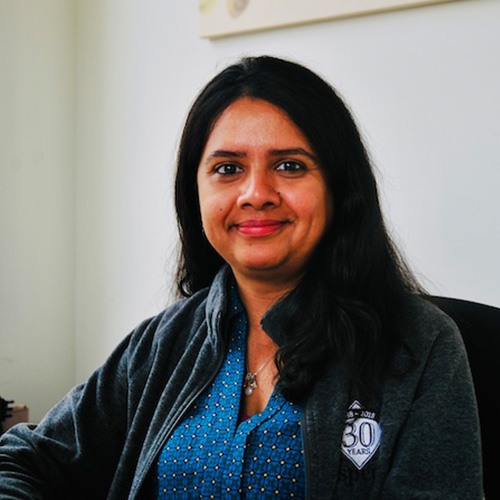
Sunita Chandrasekaran, Brookhaven National Laboratory and Lawrence Livermore National Laboratory
“ECP SOLLVE and its race to Frontier”
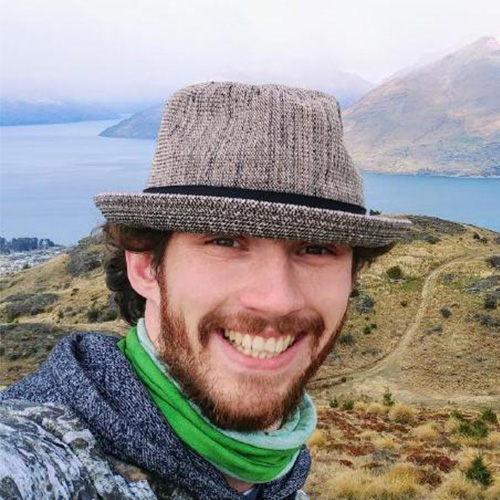
Johannes Doerfert, Brookhaven National Laboratory and Lawrence Livermore National Laboratory
» Abstract
OpenMP is a popular tool for on-node programming that is supported by a strong community of vendors, national labs, and academic groups. Several Exascale Computing Project (ECP) applications include OpenMP as part of their strategy for reaching exascale levels of performance. This talk represents the ECP SOLLVE project, where we continue to work with application partners and members of the OpenMP language committee to extend the OpenMP feature set to meet ECP application needs, especially with regard to accelerator support. This talk will present latest updates on the LLVM/Clang implementations/enhancements, their applicability on ECP applications and beyond. We will also present the current status of OpenMP offloading compiler implementations on pre-exascale and exascale system(s), their maturity and stability using our validation and verification testsuite.
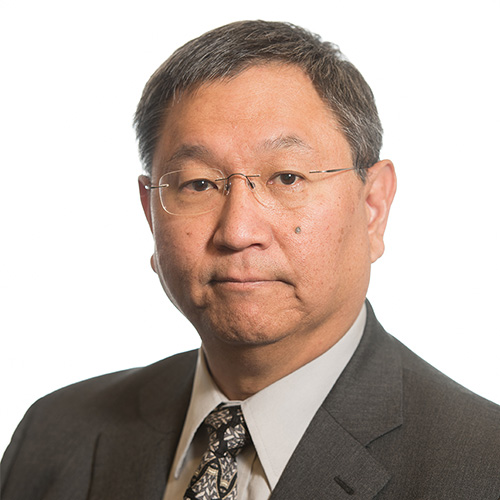
James A. Ang, Pacific Northwest National Laboratory
“New Horizons for HPC”
» Abstract
High Performance Computing is entering an era that will require significant adaptations; fundamental technologies are changing, new models of computing are emerging, and traditional ecosystems are being disrupted. The speaker describes an open innovation model, guided by HPC as a lead user and enabled by the CHIPS and Science Act, that can be an organizing principle for future computing research, bridge the valley of death with new public-private partnership models, and address the critical role of workforce development.
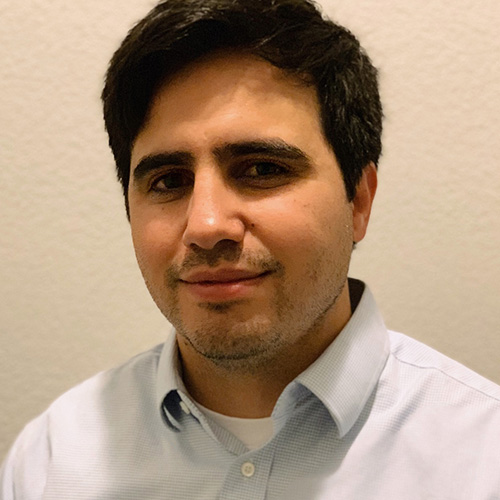
Dominic Manno, Los Alamos National Laboratory
“GUFI: The Grand Unified File Index: Performant, Secure, Accessible, and Extensible, Pick Any Four”
» Abstract
Modern data centers routinely store massive data sets resulting in millions of directories and billions of files to support thousands of simultaneous users. While existing file systems store metadata that makes it possible to query the location of specific data sets or determine which data sets are responsible for the most capacity use per user, such queries typically do not perform well at the scale of modern data center file counts. In this paper we describe the Grand Unified File Index (GUFI) that enables both data center users and data center administrators to rapidly and securely search and sift through billions of file entries to rapidly locate and characterize data sets of interest. The hierarchical indexing used by GUFI preserves access permissions so that the index can be directly securely accessed by users and also enables advanced analysis of storage system use at a large-scale data center. Further, the indexing method used in GUFII is extremely extensible, allowing data center customization trivially. Compared to the existing state-of-the-art index for file system metadata, GUFI is able to provide speedups of 1.5x to 230x for queries executed by administrators using a real file system namespace. Queries executed by users, which typically cannot rely on data center wide indexing services, see even greater speedups using GUFI.
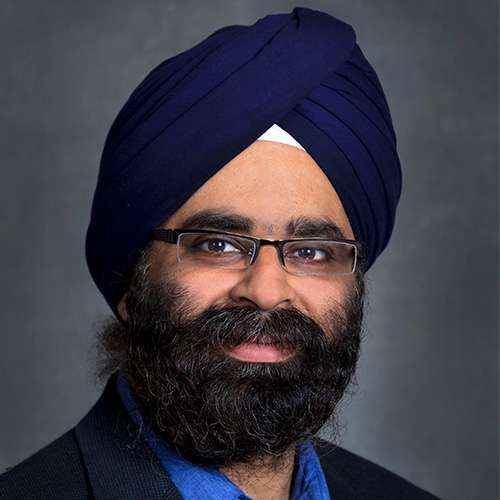
Inder Monga, Lawrence Berkeley National Laboratory
“ESnet6: How ESnet’s Next-generation Infrastructure Will Enable Integrated Research Initiative Workflows”
» Abstract
This talk will discuss the newly completed upgrade of the ESnet6 infrastructure, including the complexities of completing the project during the pandemic. ESnet Executive Director Inder Monga will provide a brief overview on the architecture of the new facility, the bandwidth deployed, the automation software stack, and the services it enables. Focus will be on recent demonstrations with laboratories that illustrate the support for the upcoming Integrated Research Initiative and how the features of ESnet6 enable that vision.
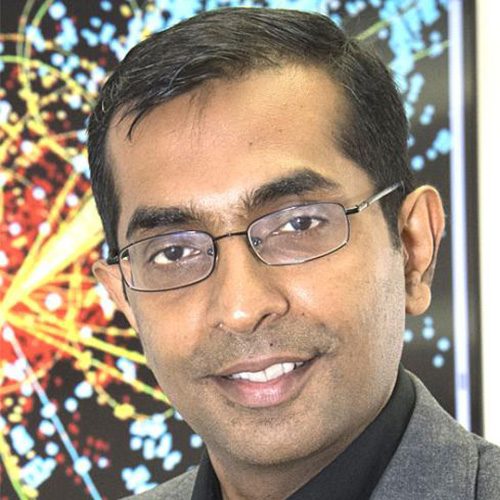
Shantenu Jha, Brookhaven National Laboratory
“ZettaWorks: Taking ExaWorks to the next frontier”
» Abstract
High-performance workflows are necessary for scientific discovery. We outline how ExaWorks is enabling workflows at extreme scales, and a vision for ExaWorks beyond exascale.
Wednesday, Nov. 16
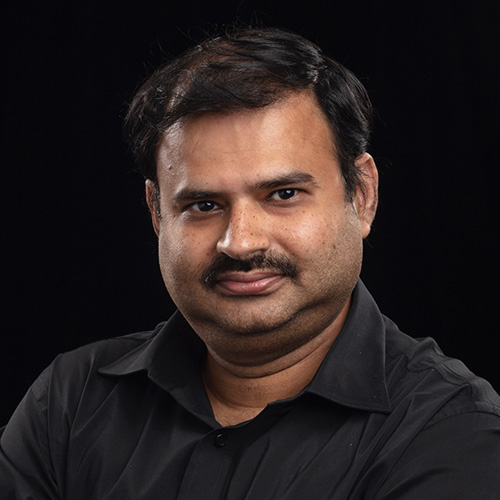
Ramakrishnan Kannan, Oak Ridge National Laboratory
“ExaFlops Biomedical Knowledge Graph Analytics”
» Abstract
We are motivated by newly proposed methods for mining large-scale corpora of scholarly publications (e.g., full biomedical literature), which consists of tens of millions of papers spanning decades of research. In this setting, analysts seek to discover relationships among concepts. They construct graph representations from annotated text databases and then formulate the relationship-mining problem as an all-pairs shortest paths (APSP) and validate connective paths against curated biomedical knowledge graphs (e.g., SPOKE). In this context, we present COAST (Exascale Communication-Optimized All-Pairs Shortest Path) and demonstrate 1.004 EF/s on 9,200 Frontier nodes (73,600 GCDs). We develop hyperbolic performance models (HYPERMOD), which guide optimizations and parametric tuning. The proposed COAST algorithm achieved the memory constant parallel efficiency of 99% in the single-precision tropical semiring. Looking forward, COAST will enable the integration of scholarly corpora like PubMed into the SPOKE biomedical knowledge graph.
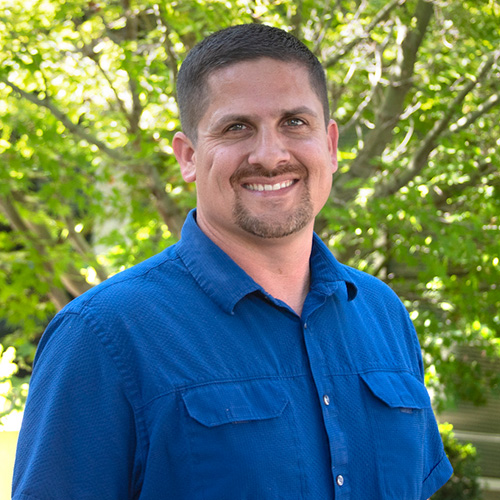
Chris DePrater, Lawrence Livermore National Laboratory
“Facilities Path to Exascale”
» Abstract
A supercomputer doesn’t just magically appear, especially one as large and as fast as Lawrence Livermore National Laboratory’s (LLNL) upcoming exascale-class El Capitan. Projected to be among the world’s most powerful supercomputers when it is deployed at LLNL in 2023, El Capitan at peak will require about as much power as a small city. Preparing the Livermore Computing Center for El Capitan and the Exascale Era of supercomputers, capable of calculations in the quintillions per second, required an entirely new way of thinking about the facility’s mechanical and electrical capabilities — a utility-scale solution. More than 15 years in planning and development, the $100 million Exascale Computing Facility Modernization (ECFM) project nearly doubles the energy capacity of the Lab’s main computing facility to 85 megawatts – enough electricity to power about 75,000 modest-sized homes. It also expands the facility’s water-cooling system capacity from 10,000 tons to 28,000 tons. The ECFM project required an extensive permitting process, coordination with local utility companies and the contributions of hundreds of people. After breaking ground in 2020, construction crews installed a 115kV transmission line, air switches, substation transformers, a switchgear, relay control enclosures, 13.8 kilovolt secondary feeders and cooling towers In an area adjacent to Building 453 — the Lab’s main computing facility. Despite the COVID-19 pandemic, the project was finished under budget and months ahead of schedule, completed on June 8, 2022. The upgrade will enable LLNL and the two other National Nuclear Security Administration (NNSA) laboratories—Los Alamos and Sandia—to use El Capitan and other next-generation supercomputers in the coming years to regularly perform the advanced modeling and simulation necessary to meet the increasingly demanding needs of NNSA’s Stockpile Stewardship Program, which ensures the safety, security and reliability of the nation’s nuclear deterrent.
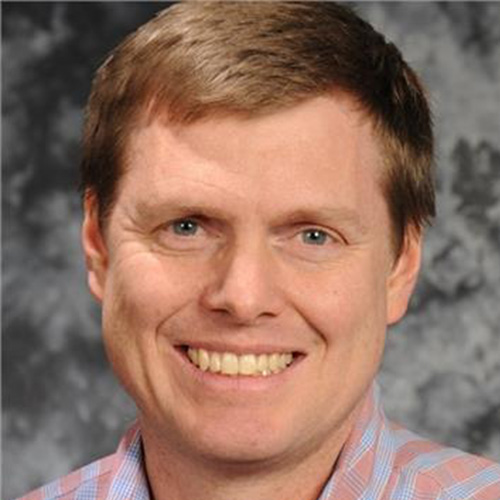
Matthew Anderson, Idaho National Laboratory
“Field programmable gate arrays in HPC workflows”
» Abstract
This talk will cover the rise in usage of field programmable gate arrays in HPC workflows and will explore several examples supporting nuclear energy research. We provide case studies comparing several different GPUs and FPGA evaluation boards deployed for emerging workflows and include power considerations.
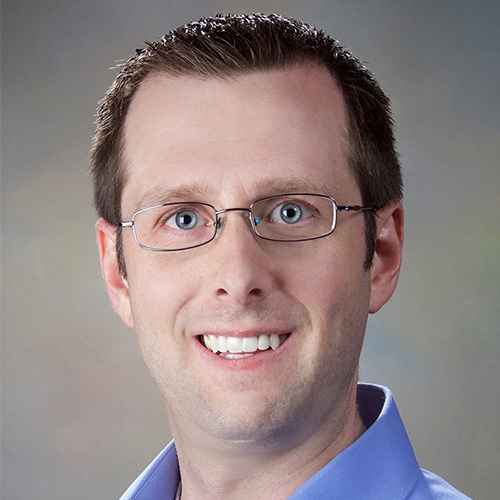
Stan Moore, Sandia National Laboratories
“Extreme-Scale Atomistic Simulations of Molten Metal Expansion”
» Abstract
For some flyers and wire vaporization experiments (for example on Sandia National Laboratories’ Z Pulsed Power Facility) the expanding material enters the liquid-vapor coexistence region. Most continuum hydrodynamics codes that model these experiments use equilibrium equations of state, assuming phase transformation kinetics are short compared to the dynamics of the simulation. If this equilibrium assumption is incorrect (i.e. the liquid-vapor transformation kinetics are long compared to the simulation dynamics), then once material enters these two-phase regions, the simulation is no longer valid. Extreme-scale molecular dynamics (MD) simulations (over a billion atoms) on NNSA’s ATS-2 Sierra supercomputer, using up to 8192 NVIDIA V100 GPUs, investigate this issue by modeling the expansion of molten supercritical material into the liquid-vapor coexistence region at the atomic level. These atomistic simulations avoid making any explicit assumptions about the material behavior (e.g. droplet formation, coalescence, break-up, surface tension, heat transfer, etc.) commonly needed for continuum models. A realistic model for aluminum has been developed using the SNAP machine learning interatomic potential in the LAMMPS MD code, trained with DFT quantum chemistry calculations. Information from these atomistic simulations can generate unprecedented insight into phase change kinetics and fluid microstructure evolution, providing a basis for improving two-phase equation-of-state models in hydrocode simulations. Optimizations of the SNAP code for GPUs over the last several years (giving over 30x speedup), and the challenge of visualizing over a billion atoms in parallel, will also be described. Sandia National Laboratories is a multimission laboratory managed and operated by National Technology and Engineering Solutions of Sandia, LLC., a wholly owned subsidiary of Honeywell International, Inc., for the U.S. Department of Energy’s National Nuclear Security Administration under contract DE-NA-0003525.
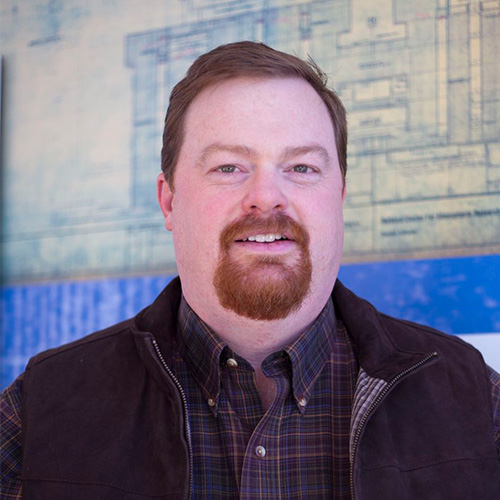
Aaron Andersen, National Renewable Energy Laboratory
“Meet Kestrel: NREL’s Third-Generation High Performance Computing System”
» Abstract
Kestrel represents the third generation HPC system to be installed in the Energy Systems Integration Facility (ESIF) at the National Renewable Energy Lab (NREL). Features of the new system will be highlighted including the application of the system to computing our energy future. ESIF as the home of the system continues as an exemplar of energy efficiency in HPC and functions as both a production computing facility and living laboratory. Recent accomplishments with respect to energy efficiency will also be presented.
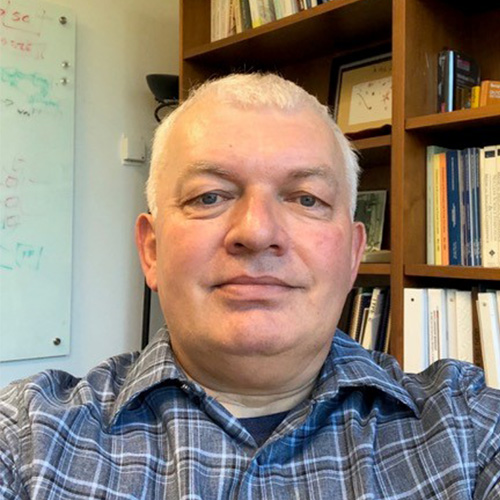
Graham Heyes, Jefferson Lab
“Computing Models for Processing Streaming Data from DOE Science”
» Abstract
The Department of Energy National Laboratories support science programs across a broad range of scientific disciplines. Scientific instruments range in scale and complexity from tabletop experiments to building sized nuclear and high energy physics detectors. In the past, the ability to transport, store, and process data constrained data rates. This resulted in data that was effectively a series snapshots capturing instants in time. The availability of high performance computing, networking, and storage, now allows continuous readout of instruments in a streaming mode, analogous to video compared with snapshots. Streaming data has the capability of capturing more science but also a significant fraction of data that is uninteresting. This presentation looks at some of the techniques and challenges associated with processing streaming data from science experiment.
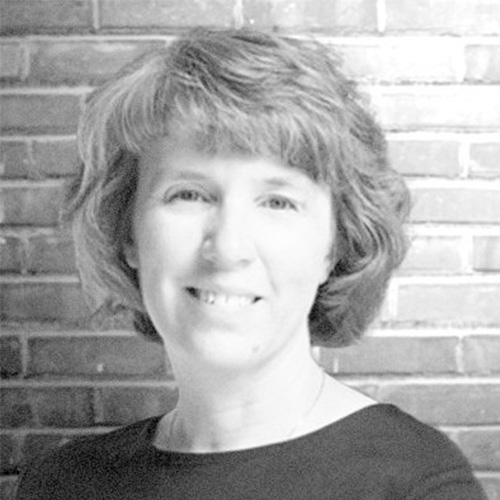
Lori Diachin, Lawrence Livermore National Laboratory
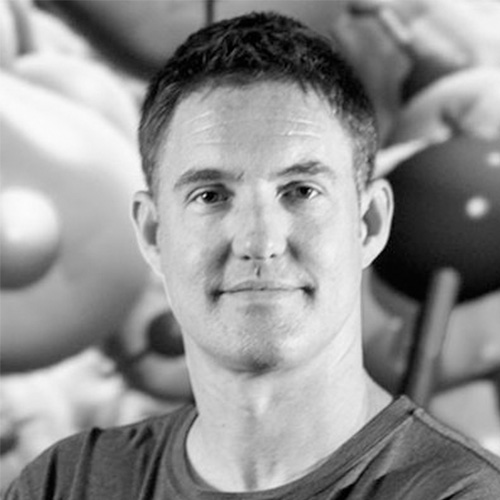
Erik Draeger, Lawrence Livermore National Laboratory
“The Exascale Computing Project”
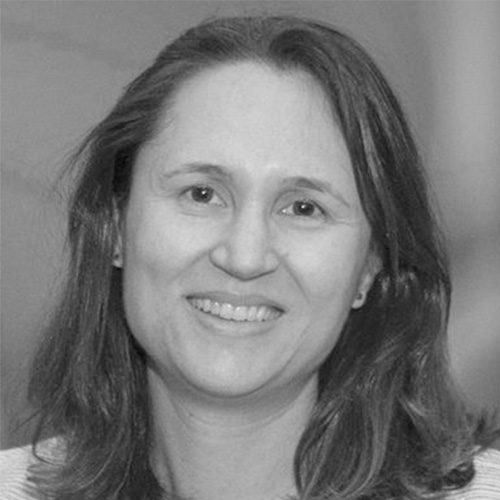
Katie Antypas, National Energy Research Scientific Computing Center
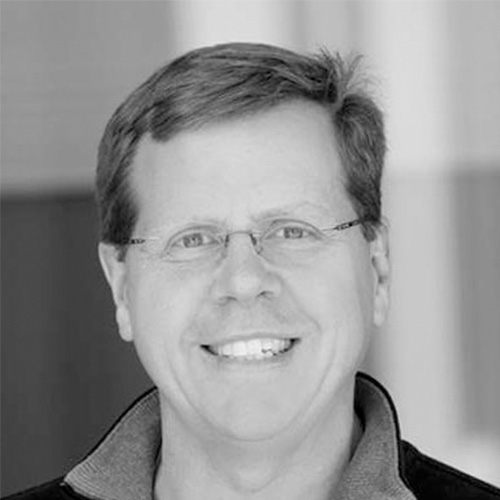
Michael Heroux, Sandia National Laboratory
» Abstract
This presentation will provide an update on the DOE Exascale Computing Project (ECP), which is developing a capable computing software ecosystem that leverages unprecedented HPC resources to solve problems by addressing predictive science capabilities in the areas of climate, energy, and human health. We will give an overview of the applications and software technologies being developed as part of the ECP, where software complexity is increasing due to disruptive changes in computer architectures and the complexities of tackling new frontiers in extreme-scale modeling, simulation, and analysis. The presentation will include examples of the challenges teams have faced in the development of new algorithms and physics capabilities that perform well on GPU accelerated node architectures. We will also describe our integrated approach to the deployment of a suite of programming models and runtimes, development tools, and libraries for math, data and visualization that comprise the Extreme-scale Scientific Software Stack (E4S). Our discussion will explain how E4S—a portfolio-driven effort in ECP to collect, test, and deliver the latest advances in open-source HPC software technologies—is helping to overcome challenges associated with using independently developed software packages together in a single application. The conclusion of this presentation will showcase some of the latest results the ECP teams have achieved in developing new capabilities in a number of application areas.
Thursday, Nov. 17
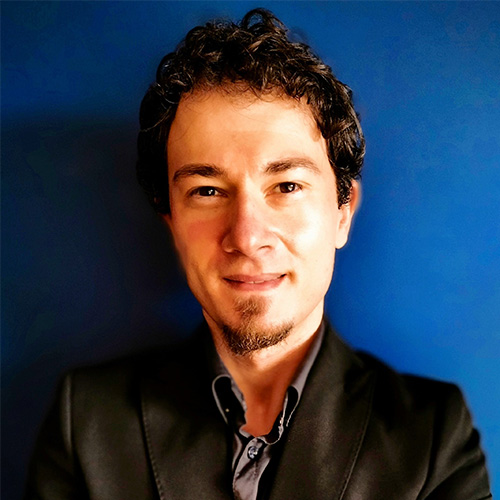
Giuseppe Barca, Ames Laboratory
“Enabling GAMESS for Exascale Quantum Chemistry”
» Abstract
Correlated electronic structure calculations enable an accurate prediction of the physicochemical properties of complex molecular systems; however, the scale of these calculations is limited by their extremely high computational cost. The Fragment Molecular Orbital (FMO) method is arguably one of the most effective ways to lower this computational cost while retaining predictive accuracy. In this lecture, a novel distributed many-GPU algorithm and implementation of the FMO method are presented. When applied in tandem with the Hartree-Fock and RI-MP2 methods, the new implementation enables correlated calculations on 623,016 electrons and 146,592 atoms in less than 45 minutes using 99.8% of the Summit supercomputer (27,600 GPUs). The implementation demonstrates remarkable speedups with respect to other current GPU and CPU codes, and excellent strong scalability on Summit achieving 94.6% parallel efficiency on 4600 nodes. This work makes feasible correlated quantum chemistry calculations on significantly larger molecular systems than before and with higher accuracy.
» 2019 Featured Speakers
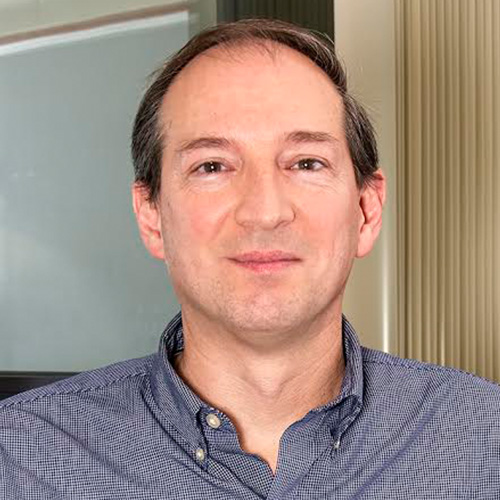
Stephane Ethier, Princeton Plasma Physics Laboratory
“High-Fidelity Whole-Device Model of Magnetically Confined Fusion Plasma”
» Abstract
The goal of this project is to develop a high-fidelity whole-device model (WDM) of magnetically confined fusion plasmas, which is urgently needed to understand and predict the performance of ITER and future next-step facilities, validated on present tokamak experiments. Guided by the understanding obtained from several fusion experiments as well as theory and simulation activities in the U.S. and abroad, ITER is expected to attain tenfold energy gain and will realize burning plasmas that are well beyond the operational regimes accessible in present and past fusion experiments. The science of fusion plasmas is inherently multi-scale in space and time, spanning several orders of magnitude in a geometrically complex configuration, and is an ideal testbed for extreme-scale computing. Our 10-year problem target on exascale computers is the high-fidelity simulation of whole-device burning plasmas applicable to a high-performance advanced tokamak regime (i.e., an ITER steady-state plasma with tenfold energy gain), integrating the effects of turbulence- and collision-induced transport, large-scale magnetohydrodynamic instabilities, energetic particles, plasma material interactions, as well as heating and current drive.
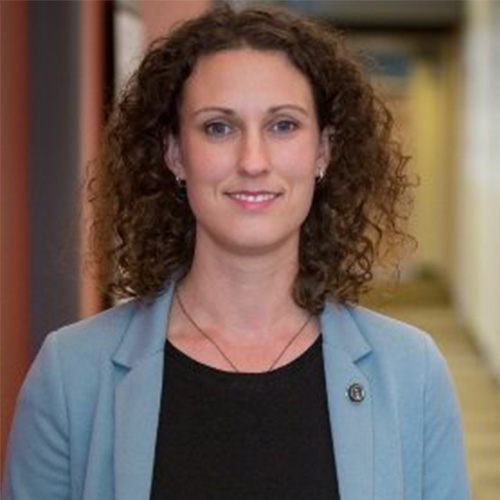
Deborah Bard, Lawrence Berkeley National Laboratory
“Cross-Facility Science: The Superfacility Model at Lawrence Berkeley National Laboratory”
» Abstract
As data sets from DOE user facilities grow in both size and complexity, there is an urgent need for new capabilities to transfer, analyze, store and curate the data to facilitate scientific discovery. DOE supercomputing facilities have begun to expand services and provide new capabilities in support of experiment workflows via powerful computing, storage, and networking systems. In this talk, I will introduce the Superfacility concept—a framework for integrating experimental and observational instruments with computational and data facilities at NERSC. I will discuss the science requirements that are driving this work, and how this translates into technical innovations in data management, scheduling, networking, and automation. In particular, I will focus on the new ways experimental scientists are accessing HPC facilities, and the implications for future system design.
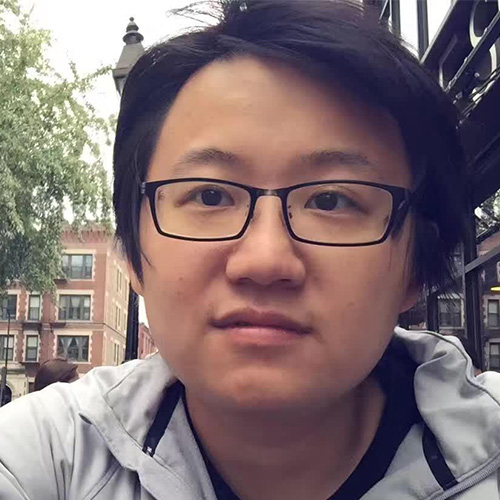
Ang Li, Pacific Northwest National Laboratory
“Online Anomalous Running Detection via Recurrent Neural Network for GPU-Accelerated HPC Machines”
» Abstract
We propose a workload classification framework that discriminates illicit computation from authorized workloads on GPU-accelerated HPC systems. As such heterogeneous systems become more powerful, they are potentially exploited by attackers to run malicious and for-profit programs that typically require extremely high computing capability to be successful. Our classification framework leverages the distinctive signatures between illicit and authorized workloads, and explores machine learning methods to learn the workloads and classify them. The framework uses lightweight, non-intrusive workload profiling to collect model input data, and explores multiple machine learning methods, particularly recurrent neural network (RNN) that is suitable for online anomalous workload detection. Evaluation results on three generations of GPU machines demonstrate that the workload classification framework can figure out the illicit unauthorized workloads with a high accuracy of over 95%. The collected dataset, detection framework, and neural network models will be released on github.
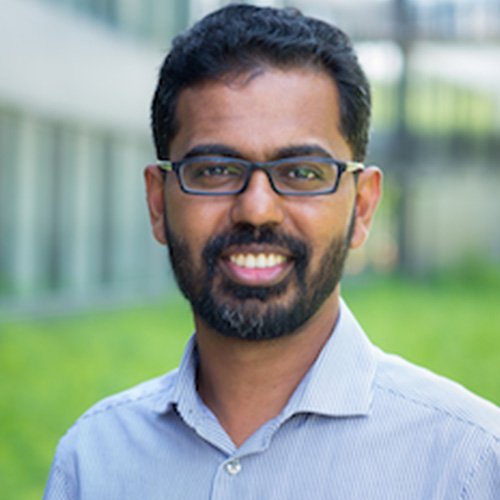
Prasanna Balaprakash, Argonne National Laboratory
“Scientific Domain-Informed Machine Learning”
» Abstract
Extracting knowledge from scientific data—produced from observation, experiment, and simulation—presents a significant hurdle for scientific discovery. As the U.S. Department of Energy (DOE) has moved toward data-driven scientific discovery, machine learning (ML) has become a critical technology in the modeling of complex phenomena in concert with current computational, experimental, and observational approaches. In the past few years, increased availability of massive data sets and growing computational power have led to breakthroughs in many scientific domains. However, development of ML systems for many scientific domains poses several challenges such as data paucity, domain-knowledge integration, and adaptability. In this talk, we will present Argonne’s work on scientific domain-informed ML approaches that seek to overcome these challenges. We will illustrate these methods using case studies on a range of DOE scientific applications. We will conclude with some exciting avenues for future research.
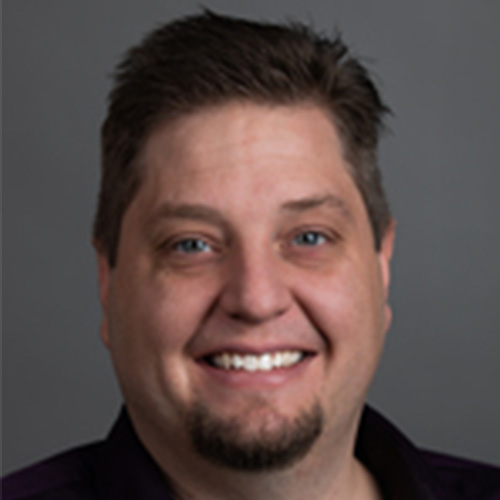
Dirk VanEssendelft, National Energy Technology Laboratory
“TensorFlow For Scientific and Engineering HPC Computations: Examples in Computational Fluid Dynamics”
» Abstract
The National Energy Technology Laboratory (NETL) has been exploring the use of TensorFlow (TF) for general scientific and engineering computations within HPC environments which might include machine learning (ML). TF has some unique capabilities in the HPC environment that could serve to reduce effort and development time. Specifically, memory management, communication, data operations, code optimization, and parallelization are handled on a wide variety of hardware in a largely automated fashion. These inherent qualities allow a practitioner to focus largely on algorithm development without necessity for deep computational science knowledge (although deep diving into TF code development can improve performance and application efficiency). NETL will provide two example cases as examples of TF capabilities for science and engineering applications in the context of computational fluid dynamics. First, NETL recently developed a novel stiff chemistry solver implemented in TF and achieved ~300× speed up over LSODA serial and ~35× speedup over LSODA parallel. Second, NETL developed a TF-based single-phase fluid solver and achieved ~3.1× improvement over 40 ranks of MPI on CPU (much higher accelerations are possible with further parallelization and better scaling is achieved when more transport equations are solved). NETL will detail early benchmarks on small to medium-scale problems and discuss how next-generation software can be significantly improved. NETL is also presenting lessons learned in short tutorial form at NVIDIA’s Expo theater as a complimentary talk.
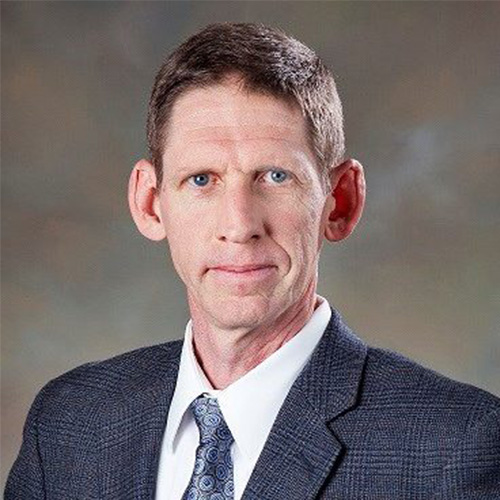
David Womble, Oak Ridge National Laboratory
“Opportunities at the Intersection of Artificial Intelligence and Science”
» Abstract
Recent impacts of artificial intelligence (AI) have been enabled by huge increases in data collection and high-performance computing. This presentation will highlight recent successes in the application and potentially disruptive opportunities of AI within the DOE mission space.
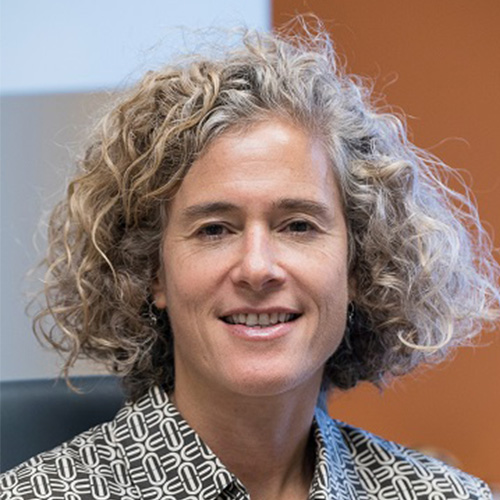
Keren Bergman, Fermi National Accelerator Laboratory
“Optically Connected Memory for High Performance Computing”
» Abstract
As the computational speed required by the cloud and high-performance computing continues to scale up, the required memory bandwidth is not keeping pace. Conventional electronic interconnects are limited by the inherent power consumption challenges of communicating high data rates over distances beyond the chip scale. Today, applications such as machine learning and deep neural networks require large memory banks to store weights and learning data. This talk will cover the opportunity offered by optically connected memory with silicon photonic links, which have the benefit of low energy per bit, small footprint, and compatibility with the current CMOS processes and ASICs.
Wednesday, Nov. 20
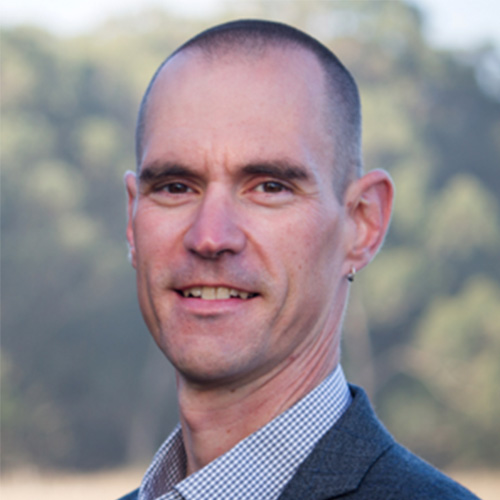
Brian Spears, Lawrence Livermore National Laboratory
“Cognitive Simulation: Integrating Large-Scale Simulations and Experiments Using Deep Learning”
» Abstract
Lawrence Livermore National Laboratory (LLNL) builds world-class predictive capabilities across a wide variety of national security missions. We continually challenge our theory-driven simulations with precision experimental data. Both simulation and experiment have become very data-rich with a complex of observables including scalars, vector-valued data, and various images. Traditional approaches can omit much of this information, making the resulting models less accurate than they otherwise could be. Today, LLNL teams are tackling this problem by developing Cognitive Simulation tools—deep learning technologies that improve predictive capabilities by effectively coupling simulation and experimental data. These CogSim techniques amplify our effective computation power, improve predictive performance, and offer new AI-driven approaches to design. To build CogSim models, we first train deep neural network models on simulation data to capture the theory implemented in advanced simulation codes. Later, we improve, or elevate, the trained models by incorporating experimental data. The training and elevation process both improves our predictive accuracy and provides a quantitative measure of uncertainty in such predictions. We will present an overview of work in this arena with specific examples from testbed research in inertial confinement fusion at the National Ignition Facility. This includes advanced deep learning architectures and methods necessary to handle rich, multimodal data and strong nonlinearities as well as techniques for reconciling these models with real experimental data. We also cover our work on enormous training sets —billions of both scalar and image observables —and models trained on them using the more than 17,000 GPUs on the Sierra supercomputer. We also describe our ongoing efforts to co-design next-generation platforms that are optimized for both precision simulation and machine learning demanded by CogSim and future applications.
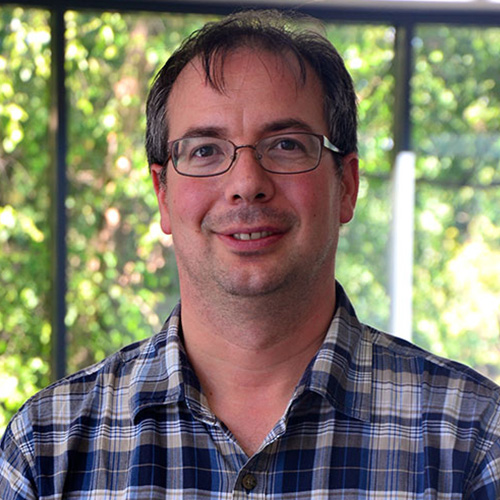
Balint Joo, Thomas Jefferson National Accelerator Facility
“HPC at Jefferson Lab for Theory and Experiment”
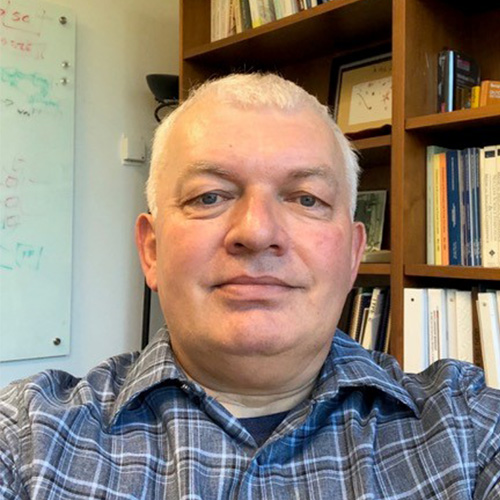
Graham Heyes, Thomas Jefferson National Accelerator Facility
» Abstract
We will discuss two of the primary computational workloads related to high performance computing at Jefferson Lab: Lattice QCD (LQCD) calculations and experimental data analysis workflows. Lattice QCD calculations are carried out in tandem with allocations at leadership facilities, with Jefferson Lab operating national shared cluster resources to provide mid-range capacity computing to the U.S. LQCD community. Jefferson Lab staff are actively engaged in software developments as part of the SciDAC-4 program and the Exascale Computing Project to exploit the most recently available compute architectures, which enable the use of both the large-scale DOE facilities as well as locally hosted cluster resources. We will detail some recent results in exploiting accelerator technologies and in the area of performance portability. In terms of data analysis, advances in all aspects of computing are beginning to make possible a new model for the analysis workflows of nuclear physics experiments, where data filtering is minimized and data is streamed in parallel through various stages of online and near-line processing, as opposed to slower models of the last 30 years, which consisted of reading the data from detectors, subjecting them to heavy filtering, and then storing the results for post-processing at a later date using thousands of individual jobs on a batch system. The new approach results in richer multi-dimensional datasets that can be made accessible for processing using grid, cloud, or leadership-class computing facilities. This is a much more responsive workflow, which leaves decisions affecting science quality as late as possible. We will provide an update on the progress of work at Jefferson Lab aimed at investigating several aspects of this new computing model. It is expected that, on the five- to ten-year timescale, streaming data readout and processing will become the norm.
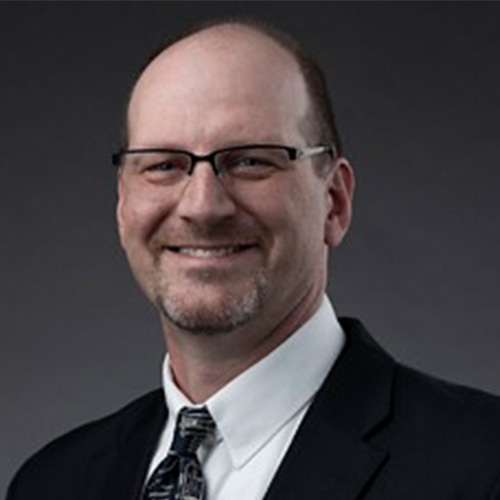
Brian Albright, Los Alamos National Laboratory
“Co-design at Extreme Scale: Finding New Efficiencies in Simulation, I/O, and Analysis”
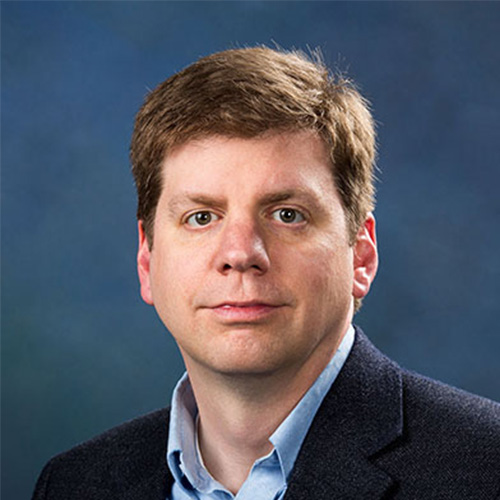
Brad Settlemyer, Los Alamos National Laboratory
» Abstract
Los Alamos National Laboratory’s (LANL’s) Vector Particle in Cell code, VPIC, has for several years been a key driver of scientific discovery in plasma physics. The per-node performance and scalability of VPIC has enabled massive simulations (up to several trillions of computational particles and hundreds of billions of computational cells) using multiple generations of supercomputers across the DOE complex. However, scientific discovery is driven not just by computational power, but also by the ability to find new insights within massive datasets. For calculations of extreme size, this can pose a profound challenge. In this talk, we describe how the ability to efficiently output and analyze data using DeltaFS is critical to the plasma physics workflow and how co-designed I/O capabilities in particular have accelerated data analysis and discovery. By combining efficient simulation and efficient data analysis within VPIC, LANL has expanded the frontiers of plasma physics and made key discoveries in a range of scientific areas, including magnetohydrodynamics, space physics, laser-plasma interaction, and the properties of high energy density matter.
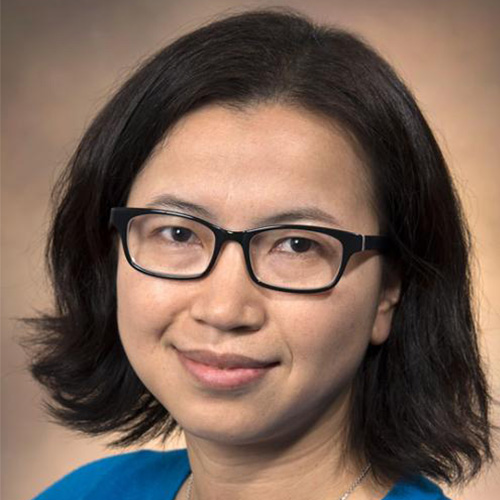
Meifeng Lin, Brookhaven National Laboratory
“High Performance Computing for Large-Scale Experimental Facilities”
» Abstract
This presentation will describe recent work in bringing high-performance computing solutions to large-scale experimental facilities, such as the National Synchrotron Light Source II (NSLS-II) at Brookhaven National Laboratory and the ATLAS experiment at CERN’s Large Hadron Collider particle accelerator. With the unprecedented amount of data continually produced at these large-scale user facilities, the need for incorporating HPC technologies and tools into experimental workflows continues to rise. Compute accelerators, such as graphics processing units (GPUs), can offer a tremendous boost to computational workloads for experiments conducted at these facilities. However, amending software to use accelerators more efficiently can be challenging. In collaboration with NSLS-II and ATLAS, Brookhaven’s Computational Science Initiative has successfully adapted some key software to use GPUs. This presentation will examine the challenges associated with porting C++- and Python-based software to GPUs and how these enhancements will impact experimental workflow approaches employed at scientific user facilities and the ways resulting data are processed.
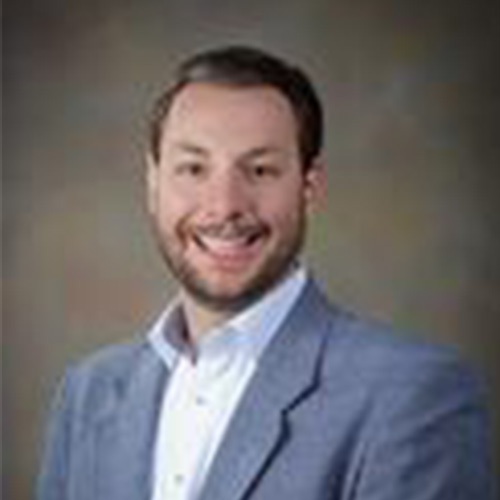
Andrew Younge, Sandia National Laboratories
“Supercontainers for HPC”
» Abstract
As the code complexity of HPC applications expands, development teams continually rely on detailed software operation workflows to enable automation of building and testing their application. These development workflows can become increasingly complex and, as a result, are difficult to maintain when the target platforms’ environments are increasing in architectural diversity and continually changing. Recently, the advent of containers in industry have demonstrated the feasibility of such workflows, and the latest support for containers in HPC environments makes them now attainable for application teams. Fundamentally, containers have the potential to provide a mechanism for simplifying workflows for development and deployment, which could improve overall build and testing efficiency for many teams. This talk introduces the Exascale Computing Project (ECP) Supercomputing Containers Project, named Supercontainers, which represents a consolidated effort across the DOE and NNSA to use a multi-level approach to accelerate adoption of container technologies for exascale. A major tenant of the project is to ensure that container runtimes are well poised to take advantage of future HPC systems, including efforts to ensure container images can be scalable, interoperable, and well integrated into exascale supercomputers across the DOE. The project focuses on foundational system software research needed for ensuring containers can be deployed at scale and provides enhanced user and developer support to ensure containerized exascale applications and software are both efficient and performant. Furthermore, these activities are conducted in the context of interoperability, effectively generating portable solutions that work for HPC applications across DOE facilities, ranging from laptops to exascale platforms.
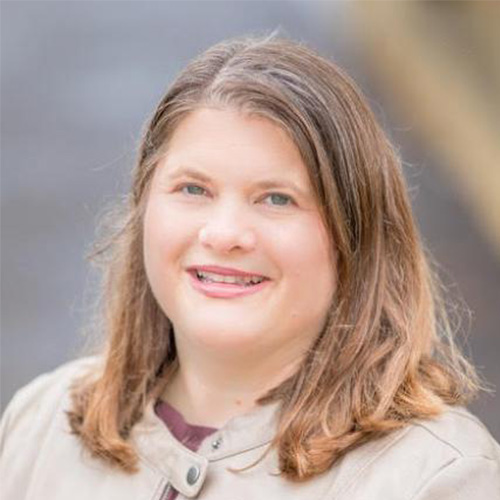
Jana Thayer, SLAC National Accelerator Laboratory
“Big Data at the Linac Coherent Light Source”
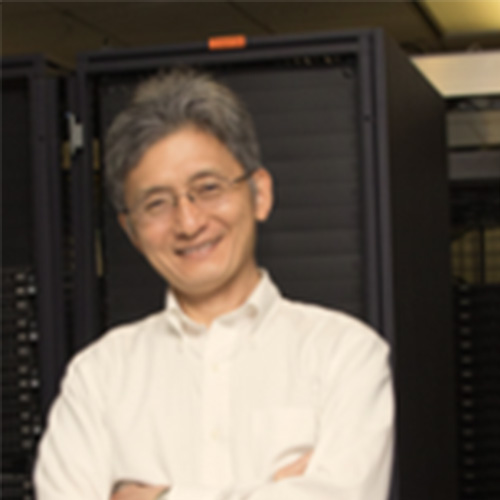
Chin Fang, SLAC National Accelerator Laboratory
» Abstract
The increase in volume and complexity of the data generated by the upcoming LCLS-II upgrade presents a considerable challenge for data acquisition, data processing, and data management. These systems face formidable challenges due to the extremely high data throughput, hundreds of GB/s to multi-TB/s, generated by the detectors at the experimental facilities and to the intensive computational demand for data processing and scientific interpretation. The LCLS Data System is a fast, powerful, and flexible architecture that includes a feature extraction layer designed to reduce the data volumes by at least one order of magnitude while preserving the science content of the data. Innovative architectures are required to implement this reduction with a configurable approach that can adapt to the multiple science areas served by LCLS. In order to increase the likelihood of experiment success and improve the quality of recorded data, a real-time analysis framework provides visualization and graphically configurable analysis of a selectable subset of the data on the timescale of seconds. A fast feedback layer offers dedicated processing resources to the running experiment to provide experimenters feedback about the quality of acquired data within minutes. We will present an overview of the LCLS Data System architecture with an emphasis on the Data Reduction Pipeline and online monitoring framework.
» 2018 Featured Speakers
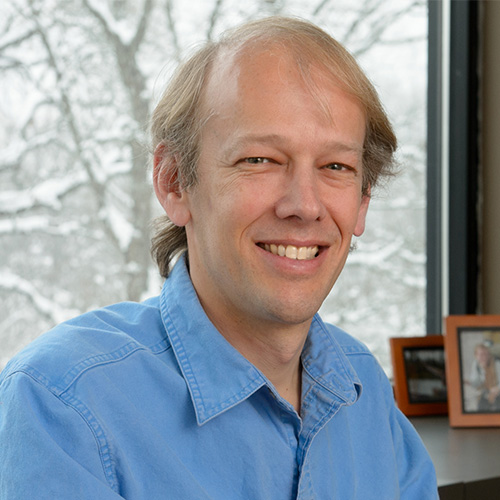
Pete Beckman, Argonne National Laboratory
“The Tortoise and the Hare: Is There Still Time for HPC to Catch Up to the Cloud in the Performance Race?”
» Abstract
Speed and scale define supercomputing. By many metrics, our supercomputers are the fastest, most capable systems on the planet. We have succeeded in deploying extreme-scale systems with high reliability, extended uptime, and large user communities. Computational science at extreme scale is leading to scientific breakthroughs. Over the past twenty years, however, the community has become overconfident in our designs for HPC system software and intelligent networking, while the cloud computing community has been steadily adding new software features and intelligent networking. From containers and virtual machines to software-defined networking and FPGAs in the fabric, the hyperscalers have been steadily moving forward building advanced systems. Has the cloud computing community already won the race? Can HPC regain leadership in the design and architecture of flexible system software and leverage containers, advanced operating systems, reconfigurable fabrics, and software-defined networking? Come learn about Argo, an operating system project for the Exascale Computing Project, how “Fluid HPC” could make large-scale system more flexible, and how the HPC community might leverage these new technologies.
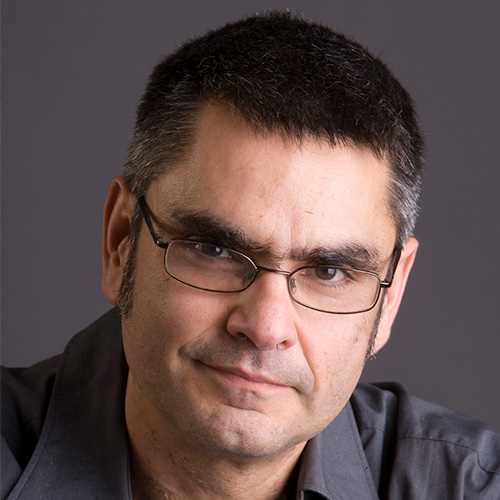
Panagiotis Spentzouris, Fermi National Accelerator Laboratory
“Fermilab’s Quantum Computing Program”
» Abstract
Fermilab’s Panagiotis Spentzouris will discuss the goals and strategy of the Fermilab Quantum Science Program, which includes simulation of quantum field theories, development of algorithms for high-energy physics computational problems, teleportation experiments and applying qubit technologies to quantum sensors in high-energy physics experiments.
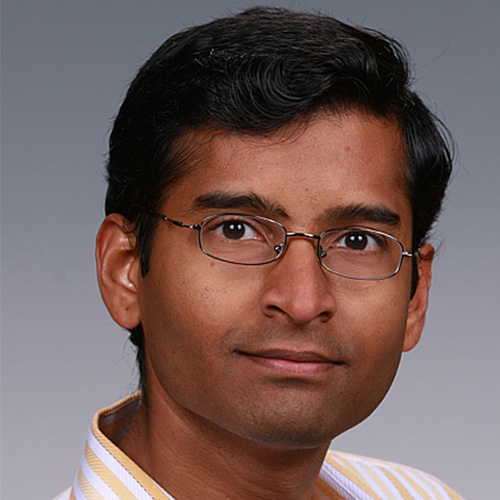
Sriram Krishnamoorthy, Pacific Northwest National Laboratory
“Intense National Focus on QIS”
» Abstract
PNNL scientist Sriram Krishnamoorthy invites you to learn how the scientific grand challenge of quantum chemistry will benefit from quantum computers. PNNL, with its depth of experience in computational chemistry, is currently exploring and designing the quantum chemistry problems that can benefit most from quantum computers. In addition, PNNL’s computer scientists and computational chemists are working closely with industry partners to jointly design the first quantum computing-based quantum chemistry calculations that surpass the limits of classical supercomputers. In this talk, Krishnamoorthy will describe these efforts and collaborations as well as other ongoing quantum computing-related activities at PNNL.
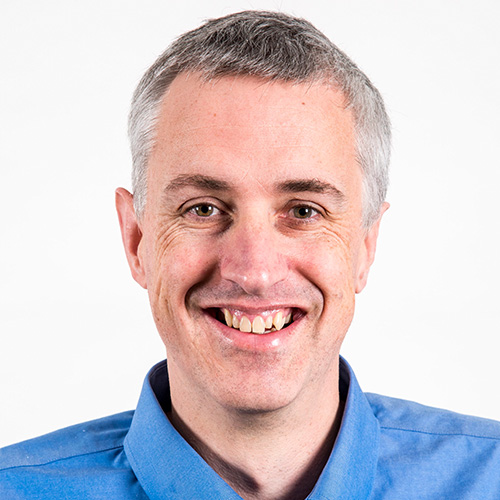
Nick Wright, Lawrence Berkeley National Laboratory
“Introducing NERSC-9, Berkeley Lab’s Next-Generation Pre-Exascale Supercomputer”
» Abstract
The NERSC-9 pre-exascale system, to be deployed in 2020, will support the broad Office of Science user community. The system is designed to support the needs of both simulations and modeling, as well as data analysis from DOE’s experimental facilities. This talk will announce and describe the NERSC-9 system for the SC18 community, including architecture features and plans for transitioning NERSC’s 7,000-member user community.
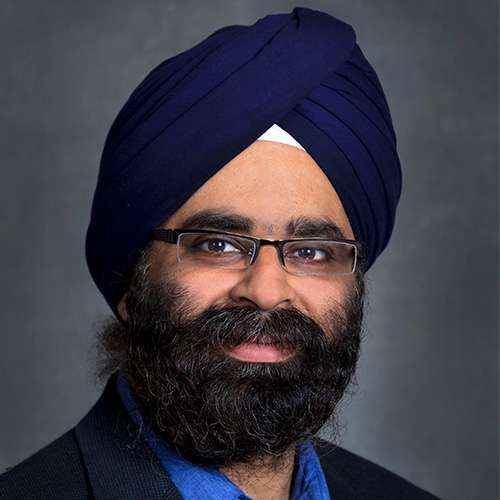
Inder Monga, Lawrence Berkeley National Laboratory
“ESnet6: Design of the Next-Generation Science Network”
» Abstract
Because of the dramatically increasing size of datasets and the need to make scientific data broadly accessible, ESnet is designing ESnet6, its next-generation network. The network will offer higher bandwidth, more growth capability, advanced features tailored for modern science and the necessary resilience to support DOE’s core research mission. The talk will discuss the conceptual ESnet6 architecture that will comprise of a programmable, scalable and resilient hollow core coupled with a flexible, dynamic and programmable services edge. ESnet6 will feature services that monitor and measure the network to make sure it is operating at peak performance. These services will also facilitate advanced cybersecurity capabilities providing the control and management needed to protect the network.
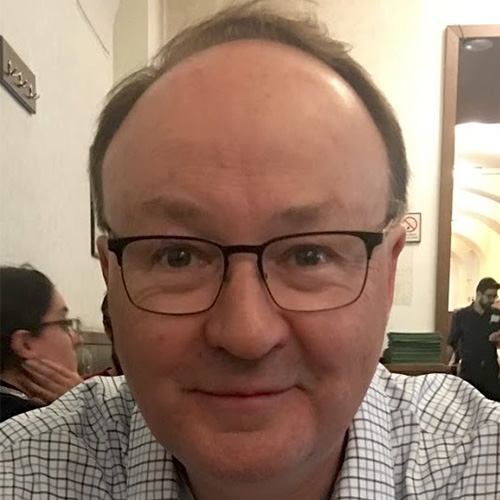
David Daniel, Los Alamos National Laboratory
“The Ristra Project: Preparing for Multi-Physics Simulation at Exascale”
» Abstract
Two key challenges on the path to efficient multi-physics simulation on exascale-class computing platforms are (a) abstracting exascale hardware from multi-physics code development, and (b) solving integral problems at multiple physical scales. Ristra, a four-year old Los Alamos project under the Advanced Technology Development and Mitigation (ATDM) sub-program of the DOE ASC program, is developing a toolkit for multi-physics code development based around a computer science interface (FleCSI) that limits the impact of disruptive computer technology on physics developers. FleCSI enables the adoption of novel programming models and data management methods to address the challenges and diversity of new technology. Simultaneously, Ristra is exploring the use of multi-scale numerical methods that offer improved physics fidelity and computing efficiency. The Ristra software architecture and progress to date will be presented, together with early results of simulations in solid mechanics and multi-scale radiation hydrodynamics.
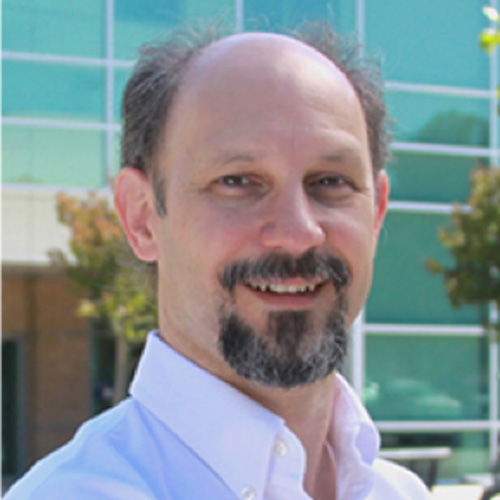
Fred Streitz, Lawrence Livermore National Laboratory
“Machine Learning and Predictive Simulation: HPC and the U.S. Cancer Moonshot on Sierra”
» Abstract
The marriage of experimental science with simulation has been a fruitful one–the fusion of HPC-based simulation and experimentation moves science forward faster than either discipline alone, rapidly testing hypotheses and identifying promising directions for future research. The emergence of machine learning at scale promises to bring a new type of thinking into the mix, incorporating data analytics techniques alongside traditional HPC to accompany experiment. I will discuss the convergence of machine learning, predictive simulation and experiment in the context of one element of the U.S. Cancer Moonshot– a multi-scale investigation of Ras biology in realistic membranes.
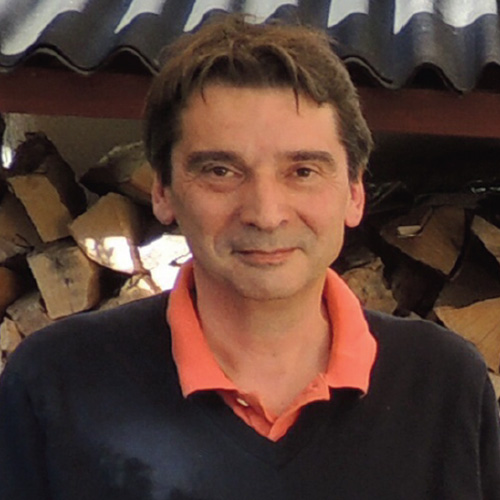
Alexei Klimentov, Brookhaven National Laboratory
“BigPanDA project. Workflow and Workload Management System for High Energy and Nuclear Physics, and for Extreme Scale Scientific Applications”
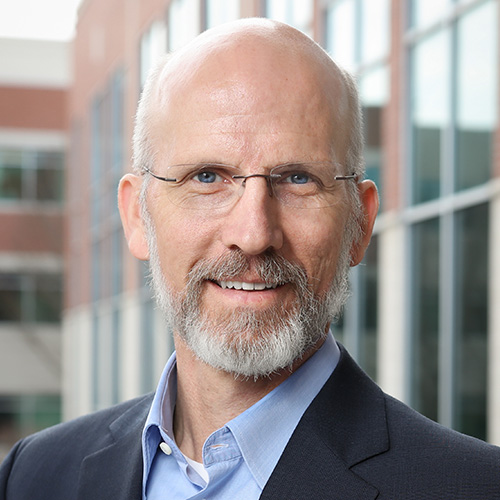
Jack Wells, Oak Ridge National Laboratory
» Abstract
The PanDA software is used for workload management on distributed grid resources by the ATLAS experiment at the LHC. An effort was launched to extend PanDA, called BigPanDA, to access HPC resources, funded by the US Department of Energy (DOE-ASCR). Through this successful effort, ATLAS today uses over 25 million hours monthly on the Titan supercomputer at Oak Ridge National Laboratory. Many challenges were met and overcome in using HPCs for ATLAS simulations. ATLAS uses two different operational modes at Titan. The traditional mode uses allocations – which require software innovations to fit the low latency requirements of experimental science. New techniques were implemented to shape large jobs using allocations on a leadership class machine. In the second mode, high priority work is constantly sent to Titan to backfill high priority leadership class jobs. This has resulted in impressive gains in overall utilization of Titan, while benefiting the physics objective s of ATLAS. For both modes, BigPanDA has integrated traditional grid computing with HPC architecture.
Wednesday, Nov. 14
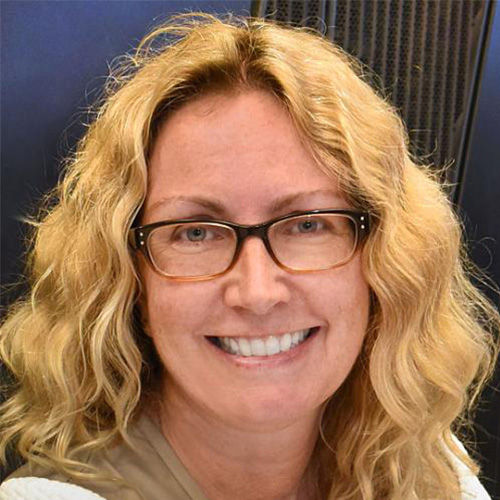
Kerstin Kleese van Dam, Brookhaven National Laboratory
“Real Time Performance Analysis of Applications and Workflows”
» Abstract
As part of the ECP CODAR project Brookhaven National Laboratory in collaboration with the Oregon Universities TAU team have developed unique capabilities to analyze, reduce and visualize single application and complete workflow performance data in-situ. The resulting tool enables the researchers to examine and explore their workflow performance as it is being executed.
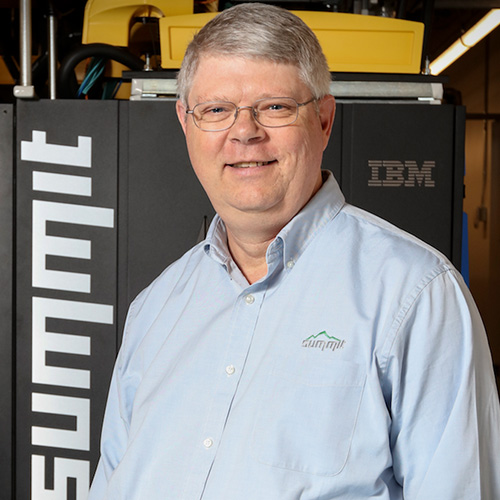
Arthur “Buddy” Bland, Oak Ridge National Laboratory
“An Overview of ORNL’s Summit Supercomputer”
» Abstract
In June 2018, U.S. Department of Energy’s Oak Ridge National Laboratory unveiled Summit as the world’s most powerful and smartest scientific supercomputer. Summit has a peak performance of 200 petaflops, and for certain scientific applications, will also be capable of more than three billion billion mixed precision calculations per second, or 3.3 exaops. Summit will provide unprecedented computing power for research in energy, advanced materials and artificial intelligence (AI), among other domains, enabling scientific discoveries that were previously impractical or impossible.
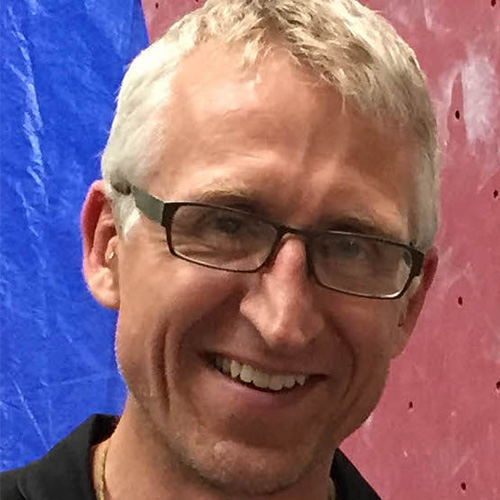
Mike Sprague, National Renewable Energy Laboratory
“ExaWind: Towards Predictive Wind Farm Simulations on Exascale Platforms”
» Abstract
This talk will describe the ExaWind Exascale Computing Project, which is in pursuit of predictive wind turbine and wind plant simulations. Predictive, physics-based high-fidelity computational models, validated with targeted experiments, provide the most efficacious path to understanding wind plant physics and reducing wind plant losses. Predictive simulations will require blade-resolved moving meshes, high-resolution grids to resolve the flow structures, hybrid-RANS/LES turbulence modeling, fluid-structure interaction, and coupling to meso-scale flows. The modeling and algorithmic pathways of ExaWind include unstructured-grid finite volume spatial discretization and pressure-projection methods for incompressible flow. The ExaWind code is Nalu-Wind, which is built on Trilinos/STK and employs the Kokkos abstraction layer for performance portability. Results will be shown for turbine simulations with the Hypre and Trilinos linear-system solver stacks with particular focus on strong scaling performance on NERSC Cori and NREL Peregrine and the underlying algebraic multigrid (AMG) preconditioners. We also describe new Hypre results on SummitDev at OLCF, and recent MW-scale single-turbine simulations under turbulent inflow.
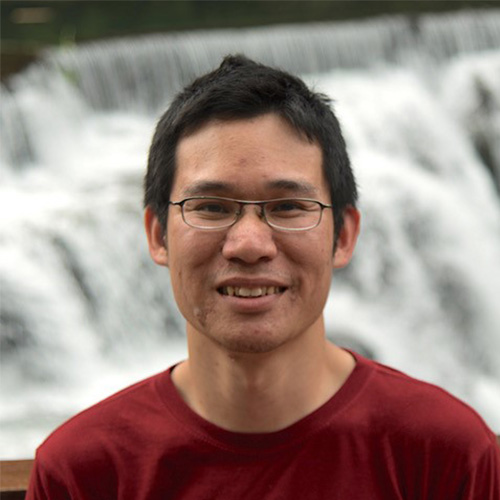
Yee Ting Li, SLAC National Accelerator Laboratory
“Hyperscale (Petabyte, Exabyte and Beyond) Data Distribution for Delivery of LCLS-II Free Electron Laser Data to Supercomputers”
» Abstract
The next generation Linear Coherent Light Source (LCLS-II) at SLAC is planned to achieve first light in 2020. The potential data rates are 1000X greater than the existing LCLS. By 2025, experimenters will need to stream data from the detectors at SLAC to DOE supercomputers at rates substantially exceeding terabits/sec. Since 2014, we have been working to create an effective solution for hyperscale data distribution. Using 5 rack-unit co-located clusters and 80Gbits/sec capacity links over a 5000mile path, we recently transferred a petabyte of encrypted data in a world-leading 29 hours. Our next steps are to transport data from SLAC to NERSC over an ESnet 100Gbps capacity link, compare software solutions and evaluate Intel Optane SSDs.
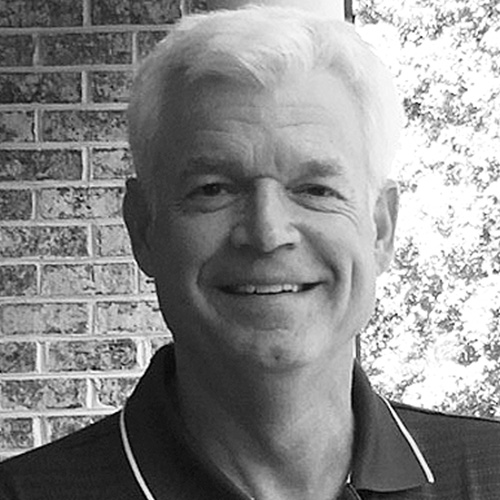
Doug Kothe, Oak Ridge National Laboratory
“Exascale Computing Project Update”
» Abstract
An update on the U.S. Department of Energy’s Exascale Computing Project – a multi-lab, 7-year collaborative effort focused on accelerating the delivery of a capable exascale computing ecosystem by 2021. The goal of the ECP is to enable breakthrough solutions that can address our most critical challenges in scientific discovery, energy assurance, economic competitiveness, and national security. The project is a joint effort of two U.S. Department of Energy (DOE) organizations: the Office of Science and the National Nuclear Security Administration (NNSA).
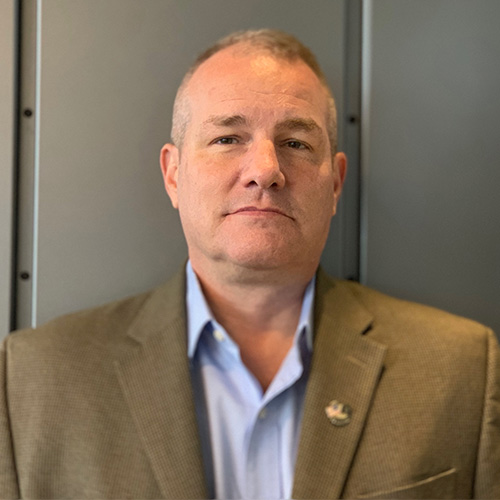
Jim Laros, Sandia National Laboratories
“Vanguard-Astra: NNSA Advanced Architecture Prototype Platform”
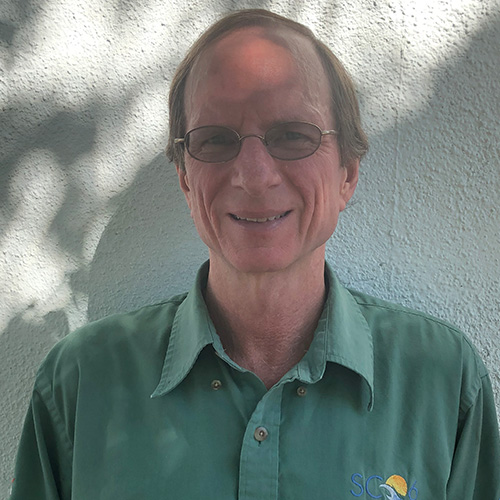
Jim Brandt, Sandia National Laboratories
“Platform Independent Run Time HPC Monitoring, Analysis, and Feedback at Any-Scale”
» Abstract
Large-scale HPC simulation applications may execute across thousands to millions of processor threads. Contention for network and/or file system resources and mismatches in processor, memory, and network resources can have significant impact on application performance. Such effects can stem from a variety of sources from manufacturing variation to resource allocation, to power and cooling variation and more. This talk presents a suite of scalable tools, developed by Sandia, to gain insight into per-instance causes of application performance degradation. We present background, architectural details and actual use case examples of monitoring sources, data, and run time analyses of that data. We also present how the output can directly inform application users and operations staff about application and system performance characteristics as well as be used to provide feedback to applications and system software components. The tools are not only useful for the insights they provide but are also fun to use and can provide hours of enjoyment for users, operations staff, and researchers trying to identify ways to architect more efficient systems/applications.
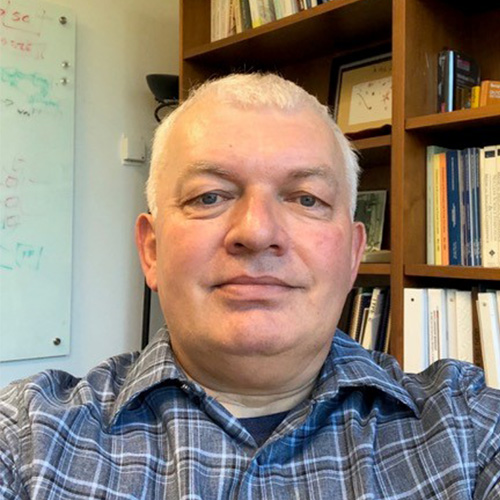
Graham Heyes, Thomas Jefferson National Accelerator Facility
“Streaming Data for Nuclear Physics Experiments”
» Abstract
The computing workflow model for most nuclear physics experiments has remained relatively unchanged for over thirty years. Data is read from detectors, heavily filtered to reduce data rate and stored. At a later date the data is retrieved and processed using thousands of individual jobs on a batch system. The final, compute intensive, processing, was performed locally since network bandwidth limited offsite data access. The whole process is slow, with weeks or months between steps, and forces the scientist to make choices in advance of data taking that affect data quality. Advances in all aspects of computing are beginning to make possible a model, new to nuclear physics, where filtering is relaxed and data is streamed in parallel through various stages of online and near line processing. This results in rich multi-dimensional datasets that can be made accessible for processing using grid, cloud, or leadership class computing facilities. This is a much more responsive workflow with minimum filtering of the raw data, which leaves decisions effecting science quality as late as possible. At Jefferson Lab several aspects of this computing model are being investigated. It is expected that, on the five to ten year timescale, streaming data readout and processing will become the norm.