Researchers from national laboratories and universities will be demonstrating new tools and technologies for accelerating data transfer, improving application performance and increasing energy efficiency in a series of demos scheduled across three days in the DOE booth at SC23 (booth 243).
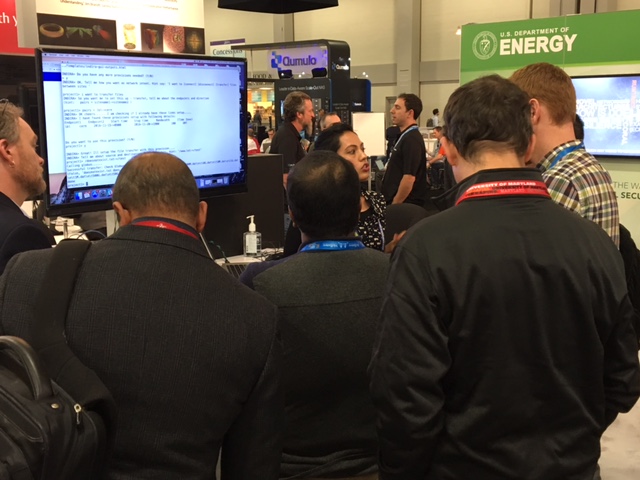
Monday, Nov. 13
Time |
Demo Station 1 |
Demo Station 2 |
7:00 p.m. |
Ann Gentile; Jim Brandt; Benjamin Schwaller; Tom Tucker (SNL) |
Prasanna Balaprakash; Feiyi Wang; Sajal Dash; Junqi Yin; Dan Lu; Ashwin Aji; Leon Song (ORNL) |
8:00 p.m. |
Ann Gentile; Jim Brandt; Benjamin Schwaller; Tom Tucker (SNL) |
Prasanna Balaprakash; Feiyi Wang; Sajal Dash; Junqi Yin; Dan Lu; Ashwin Aji; Leon Song (ORNL) |
Tuesday, Nov. 14
Time |
Demo Station 1 |
Demo Station 2 |
10:00 a.m. |
Christian Trott; Bruno Turcksin; Daniel Arndt; Nevin Liber; Rahulkumar Gayatri; Nevin Liber; Sivasankaran Rajamanickam; Luc Berger-Vergiat (ANL, LBL) |
Yao Xu; Gene Cooperman; Rebecca Hartman-Baker (LBL) |
11:00 a.m. |
Hannah Parraga, Michael Prince (ANL) |
Thomas Applencourt; Abhishek Bagusetty (ANL) |
12:00 p.m. |
Mariam Kiran; Anastasiia Butko; Ren Cooper; Imtiaz Mahmud, Nirmalendu Patra; Matthew Verlie (ORNL, LBL) |
Ann Gentile; Jim Brandt; Benjamin Schwaller; Tom Tucker (SNL) |
1:00 p.m. |
Free |
Ann Gentile; Jim Brandt; Benjamin Schwaller; Tom Tucker (SNL) |
2:00 p.m. |
Brad Richardson; Magne Haveraaen (LBL) |
Jean Luca Bez; Hammad Ather; Suren Byna; John Wu (LBL) |
3:00 p.m. |
Christine Simpson, Tom Uram, Rachana Ananthakrishnan, David Schissel, Hannah Parraga, Michael Prince (ANL) |
Ann Gentile; Jim Brandt; Benjamin Schwaller; Tom Tucker (SNL) |
4:00 p.m. |
Free |
Ann Gentile; Jim Brandt; Benjamin Schwaller; Tom Tucker (SNL) |
5:00 p.m. |
Free |
Ann Gentile; Jim Brandt; Benjamin Schwaller; Tom Tucker (SNL) |
Wednesday, Nov. 15
Time |
Demo Station 1 |
Demo Station 2 |
10:00 a.m. |
Caetano Melone (LLNL) |
Marco Minutoli (PNNL) |
11:00 a.m. |
Caetano Melone (LLNL) |
Imran Latif (BNL) |
12:00 p.m. |
Ann Gentile; Jim Brandt; Benjamin Schwaller; Tom Tucker (SNL) |
Mariam Kiran; Anastasiia Butko; Ren Cooper; Imtiaz Mahmud, Nirmalendu Patra; Matthew Verlie (ORNL, LBL) |
1:00 p.m. |
Mariam Kiran; Muneer Alshowkan; Brian Williams; Joseph Chapma (ORNL) |
Christine Simpson, Tom Uram, Rachana Ananthakrishnan, David Schissel, Hannah Parraga, Michael Prince (ANL) |
2:00 p.m. |
Yatish Kumar (LBL) |
Imran Latif (BNL) |
3:00 p.m. |
Flavio Castro; Joaquin Chung; Se-young Yu (ANL) |
Sam Wellborn; Bjoern Enders; Peter Ercius; Chris Harris; Deborah Bard (LBL) |
4:00 p.m. |
Ann Gentile; Jim Brandt; Benjamin Schwaller; Tom Tucker (SNL) |
Charles Shiflett (LBL) |
5:00 p.m. |
Ann Gentile; Jim Brandt; Benjamin Schwaller; Tom Tucker (SNL) |
Free |
Thursday, Nov. 16
Time |
Demo Station 1 |
Demo Station 2 |
10:00 a.m. |
Christian Trott; Bruno Turcksin; Daniel Arndt; Nevin Liber; Rahulkumar Gayatri; Nevin Liber; Sivasankaran Rajamanickam; Luc Berger-Vergiat (ANL, LBL) |
Christian Mayr (SNL) |
11:00 a.m. |
Flavio Castro; Joaquin Chung; Se-young Yu (ANL) |
Christian Mayr (SNL) |
12:00 p.m. |
Free |
Free |
1:00 p.m. |
Mariam Kiran; Muneer Alshowkan; Brian Williams; Joseph Chapma (ORNL) |
Free |
2:00 p.m. |
Mariam Kiran; Muneer Alshowkan; Brian Williams; Joseph Chapma (ORNL) |
Free |
2022
All listed times are in CST (Central Standard Time)
Monday, Nov. 14
Time |
Demo Station 1 |
Demo Station 2 |
7:00 p.m. |
Peer-Timo Bremer (LLNL) |
James Brandt (SNL) |
8:00 p.m. |
Peer-Timo Bremer (LLNL) |
James Brandt (SNL) |
Tuesday, Nov. 15
Time |
Demo Station 1 |
Demo Station 2 |
10:00 a.m. |
Shahzeb Siddiqui (LBNL) |
James Brandt (SNL) |
11:00 a.m. |
Sunita Chandrasekaran (BNL) |
James Brandt (SNL) |
12:00 p.m. |
Mariam Kiran (LBNL) |
Sunita Chandrasekaran (BNL) |
1:00 p.m. |
Mariam Kiran (LBNL) |
Peer-Timo Bremer (LLNL) |
2:00 p.m. |
Lee Liming (ANL) |
Peer-Timo Bremer (LLNL) |
3:00 p.m. |
Tom Scogland (LLNL) |
Peer-Timo Bremer (LLNL) |
4:00 p.m. |
Sunita Chandrasekaran (BNL) |
James Brandt (SNL) |
5:00 p.m. |
Sunita Chandrasekaran (BNL) |
James Brandt (SNL) |
Wednesday, Nov. 16
Time |
Demo Station 1 |
Demo Station 2 |
10:00 a.m. |
Hubertus (Huub) Van Dam (BNL) |
Rajkumar Kettimuthu |
11:00 a.m. |
Lee Liming (ANL) |
Rajkumar Kettimuthu |
12:00 p.m. |
Free |
Free |
1:00 p.m. |
Peer-Timo Bremer (LLNL) |
Ramesh Balakrishnan (ANL) |
2:00 p.m. |
Peer-Timo Bremer (LLNL) |
James Brandt (SNL) |
3:00 p.m. |
Peer-Timo Bremer (LLNL) |
James Brandt (SNL) |
4:00 p.m. |
Peer-Timo Bremer (LLNL) |
James Brandt (SNL) |
5:00 p.m. |
Peer-Timo Bremer (LLNL) |
James Brandt (SNL) |
Thursday, Nov. 17
Time |
Demo Station 1 |
Demo Station 2 |
10:00 a.m. |
Ezra Kissel and Charles Shiftlett (LBNL) |
James Brandt (SNL) |
11:00 a.m. |
Free |
James Brandt (SNL) |
12:00 p.m. |
Free |
Free |
1:00 p.m. |
Free |
Ramesh Balakrishnan (ANL) |
2:00 p.m. |
Free |
Ramesh Balakrishnan (ANL) |
2021
All listed times are in CST (Central Standard Time)
Tuesday, Nov. 16
10 a.m.
Anees Al Najjar (ORNL) – “Demonstrating the Functionalities of Virtual Federated Science Instrument Environment (VFSIE)”
11 a.m.
Shahzeb Siddiqui (LBNL) – “Building a Spack Pipeline in Gitlab”
12 p.m.
Joseph Insley (ANL and collaborators) – “Intel® oneAPI Rendering Toolkit: Interactive Rendering for Science at Scale”
1 p.m.
Mekena Metcalf, Mariam Kiran, and Anastasiia Butko (LBNL) – “Towards Autonomous Quantum Network Control”
2 p.m.
Laurie Stephey (LBNL), Jong Choi (ORNL), Michael Churchill (PPPL), Ralph Kube (PPPL), Jason Wang (ORNL) – “Streaming Data for Near Real-Time Analysis from the KSTAR Fusion Experiment to NERSC”
3 p.m.
Kevin Harms (ANL and collaborators) – “DAOS + Optane For Heterogenous APPs”
Wednesday, Nov. 17
10 a.m.
Narasinga Rao Miniskar and Aaron Young (ORNL) – “An Efficient FPGA Design Environment for Scientific Machine Learning”
11 a.m.
Pieter Ghysels (LBNL) – “Preconditioning Large Scale High-Frequency Wave Equations with STRUMPACK and ButterflyPACK”
12 p.m.
Mariam Kiran, Nicholas Buraglio, and Scott Campbell (LBNL) – “Hecate: Towards Self-Driving Networks in the Real World”
2 p.m.
Bjoern Enders (LBNL) – “Supporting Data Workflows with the NERSC Superfacility API”
Thursday, Nov. 18
10 a.m.
Ezra Kissel (LBNL) – “Janus: High-Performance DTN-as-a-Service”
11 a.m.
Rajkumar Kettimuthu, Joaquin Chung, and Aniket Tekawade (ANL) – “AI-Steer: AI-Driven Online Steering of Light Source Experiments + SciStream: Architecture and Toolkit for Data Streaming between Federated Science Instruments”
12 p.m.
Prasanna Balaprakash (ANL) – “DeepHyper: Scalable Neural Architecture and Hyperparameter Search for Deep Neural Networks”
2019
Tuesday, Nov. 19
Demo Station 1
We demonstrate progress and share findings from a federated Distributed Computing and Data Ecosystem (DCDE) pilot that incorporates tools, capabilities, services, and governance policies aiming to enable researchers across DOE science laboratories to seamlessly use cross-lab resources (i.e., scientific instruments, local clusters, large facilities, storage, enabling systems software, and networks). This pilot aims to present small research teams a range of distributed resources through a coherent and simple set of interfaces, to allow them to establish and manage experimental and computational pipelines and the related data lifecycle. Envisioned as a cross-lab environment, a DCDE would eventually be overseen by a governing body that includes the relevant stakeholders to create effective use and participation guidelines.
The Kokkos C++ Performance Portability Ecosystem is a production-level solution for writing modern C++ applications in a hardware-agnostic way. It is part of the U.S. Department of Energy’s Exascale Computing Project—the leading effort in the U.S. to prepare the HPC community for the next generation of supercomputing platforms. The Ecosystem consists of multiple libraries addressing the primary concerns for developing and maintaining applications in a portable way. The three main components are the Kokkos Core Programming Model; the Kokkos Kernels Math Libraries; and the Kokkos Profiling, Debugging, and Tuning Tools. Led by Sandia National Laboratories, the Kokkos team includes developers at five DOE laboratories.
Large-scale experimental science workflows require support for a unified, interactive, real-time platform that can manage a distributed set of resources connected to HPC systems. Here we demonstrate how the Jupyter platform plays a key role in this space—it provides the ease of use and interactivity of a web science gateway while allowing scientists to build custom, ad-hoc workflows in a composable way. Using real-world use cases from the National Center for Electron Microscopy and the Advanced Light Source, we show how Jupyter facilitates interactive analysis of data at scale on NERSC HPC resources.
Demo Station 2
Predicting traffic on network links can help engineers estimate the percentage bandwidth that will be utilized. Efficiently managing this bandwidth can allow engineers to have reliable file transfers and run networks hotter to send more data on current resources. Toward this end, ESnet researchers are developing advanced deep learning LSTM-based models as a library to predict network traffic for multiple future hours on network links. In this demonstration, we will show traffic peak predictions multiple hours into the future on complicated network topologies such as ESnet. We will also demonstrate how this can be used to configure network transfers to optimize network performance and utilize underused links.
Since cinema databases were first introduced in 2016, the ability for simulations and tools to produce them and viewers to explore them have improved. We will demonstrate ParaView Cinema Database creation and a number of viewers for exploring different types of cinema databases.
Modern scientific instruments are acquiring data at ever-increasing rates, leading to an exponential increase in the size of data sets. Taking full advantage of these acquisition rates will require corresponding advancements in the speed and efficiency of data analytics and experimental control. A significant step forward would come from automatic decision-making methods that enable scientific instruments to autonomously explore scientific problems —that is, to intelligently explore parameter spaces without human intervention, selecting high-value measurements to perform based on the continually growing experimental data set. Here, we develop such an autonomous decision-making algorithm based on Gaussian process regression that is physics-agnostic, generalizable, and operates in an abstract multi-dimensional parameter space. Our approach relies on constructing a surrogate model that fits and interpolates the available experimental data and is continuously refined as more data is gathered. The distribution and correlation of the data is used to generate a corresponding uncertainty across the surrogate model. By suggesting follow-up measurements in regions of greatest uncertainty, the algorithm maximally increases knowledge with each added measurement. This procedure is applied repeatedly, with the algorithm iteratively reducing model error and thus efficiently sampling the parameter space with each new measurement that it requests. The method was already used to steer several experiments at various beam lines at NSLS II and ALS. The results have been astounding; experiments that were only possible through constant monitoring by an expert were run entirely autonomously, discovering new science along the way.
Wednesday, Nov. 20
Demo Station 1
Advances in detector technologies enable increasingly complex experiments and more rapid data acquisition for experiments carried on synchrotron light sources. The data generation rates, coupled with long experimentation times, necessitate the real-time analysis and feedback for timely insights about experiments. However, the computational demands for timely analysis of high-volume and high-velocity experimental data typically exceed the locally available resources and require utilization of large-scale clusters or supercomputers. In this demo, we will simulate experimental data generation at Advanced Photon Source beamlines and stream data to Argonne Leadership Computing Facility for real-time image reconstruction. The reconstructed images data will then be denoised and enhanced using machine learning techniques and streamed back for 2D or 3D volume visualization.
The Kokkos C++ Performance Portability Ecosystem is a production-level solution for writing modern C++ applications in a hardware-agnostic way. It is part of the U.S. Department of Energy’s Exascale Computing Project—the leading effort in the U.S. to prepare the HPC community for the next generation of supercomputing platforms. The Ecosystem consists of multiple libraries addressing the primary concerns for developing and maintaining applications in a portable way. The three main components are the Kokkos Core Programming Model; the Kokkos Kernels Math Libraries; and the Kokkos Profiling, Debugging, and Tuning Tools. Led by Sandia National Laboratories, the Kokkos team includes developers at five DOE laboratories.
TAU Performance System® is a portable profiling and tracing toolkit for performance analysis of parallel programs written in Fortran, C, C++, Python, and Java. It is capable of gathering performance information through instrumentation of functions, methods, basic blocks, and statements. TAU supports HPC runtimes including MPI, pthread, OpenMP, CUDA, OpenCL, OpenACC, HIP, and Kokkos. All C++ language features are supported including templates and namespaces. The API also provides selection of profiling groups for organizing and controlling instrumentation. The instrumentation can be inserted in the source code using an automatic instrumentor tool based on the Program Database Toolkit (PDT), dynamically using DyninstAPI, at runtime using library preloading using tau_exec, and interpreter level instrumentation using Python, or even manually using the instrumentation API. TAU internally uses OTF2 to generate traces that may be visualized using the Vampir toolkit. TAU’s profile visualization tool, paraprof, provides graphical displays of all the performance analysis results in aggregate and single node/context/thread forms. The user can quickly identify sources of performance bottlenecks in the application using the graphical interface. In addition, TAU can generate event traces that can be displayed with the Vampir, Paraver or JumpShot trace visualization tools. TAU provides integrated instrumentation, measurement, and analysis capabilities in a cross-platform tool suite, plus additional tools for performance data management, data mining, and interoperation. The TAU project has developed strong interactions with the ASC/NNSA, ECP, and SciDAC. TAU has been ported to the leadership-class facilities at ANL, ORNL, LLNL, Sandia, LANL, and NERSC, including GPU Linux clusters, IBM, and Cray systems. TAU Commander simplifies the workflow of TAU and provides support for experiment management, instrumentation, measurement, and analysis tools.
Large-scale experimental science workflows require support for a unified, interactive, real-time platform that can manage a distributed set of resources connected to HPC systems. Here we demonstrate how the Jupyter platform plays a key role in this space—it provides the ease of use and interactivity of a web science gateway while allowing scientists to build custom, ad-hoc workflows in a composable way. Using real-world use cases from the National Center for Electron Microscopy and the Advanced Light Source, we show how Jupyter facilitates interactive analysis of data at scale on NERSC HPC resources.
Demo Station 2
During HPC system acquisition, significant consideration is given to the desired performance, which, in turn, drives the selection of processing components, memory, high-speed interconnects, file systems, and more. The achieved performance, however, is highly dependent on operational conditions and which applications are being run concurrently along with associated workflows. Therefore, the performance bottleneck discovery and assessment process are critical to performance optimization. HPC system monitoring has been a long-standing need for administrators to assess the health of their systems, detect abnormal conditions, and take informed actions when restoring system health. Moreover, users strive to understand how well their jobs run and what the architecture limits that restrict the performance of their jobs are. In this demonstration, we will present new features of a large-scale HPC monitoring framework called Lightweight Distributed Metric Service (LDMS). We will demonstrate a new anomaly detection capability that uses machine learning in conjunction with monitoring data to analyze system and application health in advanced HPC systems. We will also demonstrate a Top-down Microarchitecture Analysis (TMA) implementation that uses hardware performance counter data and computes a hierarchical classification of how an execution has utilized various parts of the hardware architecture. Our new Distributed Scalable Object Store (DSOS) will be presented and demonstrated, along with performance numbers that enable comparison with other current database technologies.
Predicting traffic on network links can help engineers estimate the percentage bandwidth that will be utilized. Efficiently managing this bandwidth can allow engineers to have reliable file transfers and run networks hotter to send more data on current resources. Toward this end, ESnet researchers are developing advanced deep learning LSTM-based models as a library to predict network traffic for multiple future hours on network links. In this demonstration, we will show traffic peak predictions multiple hours into the future on complicated network topologies such as ESnet. We will also demonstrate how this can be used to configure network transfers to optimize network performance and utilize underused links.
Workflows that span instrument and computational facilities are driving new requirements in automation, data management and transfer, and development of science gateways. NERSC has initiated engagements with a number of projects that drive these emerging needs and is developing new capabilities to meet them. Learn more about NERSC’s plans and see demonstrations of a new API for interacting with NERSC systems and demonstrations of Spin, a Docker-based science gateway infrastructure.
TAU Performance System® is a portable profiling and tracing toolkit for performance analysis of parallel programs written in Fortran, C, C++, Python, and Java. It is capable of gathering performance information through instrumentation of functions, methods, basic blocks, and statements. TAU supports HPC runtimes including MPI, pthread, OpenMP, CUDA, OpenCL, OpenACC, HIP, and Kokkos. All C++ language features are supported including templates and namespaces. The API also provides selection of profiling groups for organizing and controlling instrumentation. The instrumentation can be inserted in the source code using an automatic instrumentor tool based on the Program Database Toolkit (PDT), dynamically using DyninstAPI, at runtime using library preloading using tau_exec, and interpreter level instrumentation using Python, or even manually using the instrumentation API. TAU internally uses OTF2 to generate traces that may be visualized using the Vampir toolkit. TAU’s profile visualization tool, paraprof, provides graphical displays of all the performance analysis results in aggregate and single node/context/thread forms. The user can quickly identify sources of performance bottlenecks in the application using the graphical interface. In addition, TAU can generate event traces that can be displayed with the Vampir, Paraver or JumpShot trace visualization tools. TAU provides integrated instrumentation, measurement, and analysis capabilities in a cross-platform tool suite, plus additional tools for performance data management, data mining, and interoperation. The TAU project has developed strong interactions with the ASC/NNSA, ECP, and SciDAC. TAU has been ported to the leadership-class facilities at ANL, ORNL, LLNL, Sandia, LANL, and NERSC, including GPU Linux clusters, IBM, and Cray systems. TAU Commander simplifies the workflow of TAU and provides support for experiment management, instrumentation, measurement, and analysis tools.
2018
Tuesday, Nov. 13
Demo Station 1
As part of the ECP CODAR project, Brookhaven National Laboratory, in collaboration with the Oregon Universities TAU team, has developed unique capabilities to analyze, reduce and visualize single application and complete workflow performance data in-situ. The resulting tool enables the researchers to examine and explore their workflow performance as it is being executed.
In DOE research communities, the emergence of distributed, extreme-scale science applications is generating significant challenges regarding data transfer. The data transfer challenges of the extreme-scale era are typically characterized by two relevant dimensions: high-performance challenges and time-constraint challenges. To meet these challenges, DOE’s ASCR office has funded Fermilab and Oak Ridge National Laboratory to collaboratively work on the BigData Express project (http://bigdataexpress.fnal.gov). BigData Express seeks to provide a schedulable, predictable, and high-performance data transfer service for DOE’s large-scale science computing facilities and their collaborators. Software defined technologies are key enablers for BigData Express. In particular, BigData Express makes use of software-defined networking (SDN) and software-defined storage (SDS) to develop a data-transfer-centric architecture to optimally orchestrate the various resources in an end-to-end data transfer loop. With end-to-end integration and coordination, network congestion and storage IO contentions are effectively reduced or eliminated. As a result, data transfer performance is significantly improved. BigData Express has recently gained growing attention in the communities. The BigData Express software is being deployed at multiple research institutions, which include UMD, StarLight, FNAL, KISTI (South Korea), UVA, and Ciena. Meanwhile, the BigData Express research team is collaborating with StarLight to deploy BigData Express at various research platforms, including Pacific Research Platform, National Research Platform, and Global Research Platform. It is envisioned that we are working toward building a multi-domain and multi-tenant software defined infrastructure (SDI) for high-performance data transfer.In this demo, we use BDE software to demonstrate bulk data movement over wide area networks. Our goal is to demonstrate that BDE can successfully address the high-performance and time-constraint challenges of data transfer to support extreme-scale science applications.
ParaView will be running with large data on a remote cluster at Sandia National Laboratories.
TAU Performance System is a portable profiling and tracing toolkit for performance analysis of parallel programs written in Fortran, C, C++, Python, and Java.It is capable of gathering performance information through instrumentation of functions, methods, basic blocks, and statements. All C++ language features are supported including templates and namespaces. The API also provides selection of profiling groups for organizing and controlling instrumentation. The instrumentation can be inserted in the source code using an automatic instrumentor tool based on the Program Database Toolkit (PDT), dynamically using DyninstAPI, at runtime using library preloading using tau_exec, and interpreter level instrumentation using Python, or even manually using the instrumentation API. TAU internally uses OTF2 to generate traces that may be visualized using the Vampir toolkit.
X-ray ptychography is an important tool for reconstructing high-resolution specimen images from the scanning diffraction measurements. As an inverse problem, while there exists no unique solution to the ptychographical reconstructions, one of the best working approaches is the so-called difference map algorithm, based on which the illumination and object profiles are updated iteratively with the amplitude of their product constrained by the measured intensity at every iteration. Although this approach converges very fast (typically less than 100 iterations), it is a computationally intensive task and often requires several hours to retrieve the result on a single CPU, which is a disadvantage especially for beamline users who have limited access time. We accelerate this ptychography calculation by utilizing multiple GPUs and MPI communication. We take the scatter-and-gather approach by splitting the measurement data and sending each portion to a GPU node. Since data movement between the host and the device is expensive, the data is kept and the calculation is performed entirely on GPU, and only the updated probe and object are broadcasted at the end of each iteration. We show that our program has an excellent constant weak-scaling and enables users to obtain the results on the order of sub-minutes instead of hours, which is crucial for visualization, real-time feedback and efficient adjustment of experiments. This program is already put in production in the HXN beamline at NSLS-II, and a graphical user interface is also provided.
As the volume and velocity of data generated by experiments continues to increase, we find the need to move data analysis and reduction operations closer to the source of the data to reduce the burden on existing HPC facilities that threaten to be overrun by the surge of experimental and observational data. Furthermore, remote facilities, including astronomy observatories, particle accelerators such as SLAC LCLS-II, etc. producing data do not necessarily have dedicated HPC facilities on-site. These remote sites are often power or space constrained, making the construction of a traditional data center or HPC facility unreasonable. Further complicating these scenarios, each experiment often needs a blend of specialized and programmable hardware that is closely tied to the needs of the individual experiment. We propose a hardware generation methodology based on open-source components to rapidly design and deploy these data filtering and analysis computing devices. He re we demonstrate a potential near-sensor, real-time, data processing solution developed using an innovative open-source hardware generation technique allowing potentially more effective use of experimental devices, such as electron microscopy.
The ongoing 4-year collaboration between ORNL and Appentra is enabling the development of new tools using the Parallelware technology to address the needs of leading HPC centers. Firstly, we will present Parallelware Trainer (https://www.appentra.com/products/parallelware-trainer/), a scalable interactive teaching environment tool for HPC education and training targeting OpenMP and OpenACC for multicores and GPUs. And secondly, we will present Parallelware Analyzer, a new command-line reporting tool aimed at improving the productivity of HPC application developers. The tool has been selected as an SC18 Emerging Technology, and we will demonstrate how it can help to facilitate the analysis of data layout and data scoping across procedure boundaries. The presentation with make enphasis on the new and upcoming technical features of the underlying Parallelware technology.
Demo Station 2
This demonstration will provide a brief overview of a suite of HPC monitoring and analysis tools developed in collaboration between Sandia National Laboratories (SNL), Los Alamos National Laboratories (LANL), and Open Grid Computing (OGC). The demonstration will provide a highlight overview of: 1) our Lightweight Distributed Metric Service (LDMS) for data collection, transport, and storage (including use case examples of system and job based analyses with visualization) and 2) Baler tool for mapping log messages into patterns and performing a variety of pattern based analyses. For additional information about our suite of HPC monitoring and analysis tools please email ovis-help@sandia.gov or visit http://www.opengridcomputing.com/sc18
Accelerator such as GPU play an essential role in today’s HPC systems. However, programming accelerators is often challenging. One of the most difficult part is to manage accelerator memory. OpenMP has supported accelerator offloading for a while and is gaining more and more usage. Currently, it requires users to explicitly manage memory mapping between host and accelerator, which needs a large amount of efforts from programmers. In OpenMP 5.0, user defined mapper and the support of unified memory are introduced to facilitate accelerator data management. We will introduce how to utilize these features to improve applications in this demo.
In situ visualization and analysis is an important component of the path to exascale computing. Coupling simulation codes directly to analysis codes reduces their I/O while increasing the temporal fidelity of the analysis. SENSEI, a light weight in situ frame work, gives simulations access to a diverse set of analysis back ends through a simple API and data model. SENSEI currently supports ParaView Catalyst, VisIt Libsim, ADIOS, Python, and VTK-m based back ends and is easy to extend. In this presentation we introduce SENSEI and demonstrate its use with the IAMR an AMReX based compressible Navier Stokes simulation code.
The trends in high performance computing, where far more data can be computed that can ever be stored, have made on line processing techniques an important area of research and development. In this demonstration, we show on line visualization of data from XGC1, a particle-in-cell code used to study the plasmas in fusion tokamak devices. We use the ADIOS software framework for the on line data management and use the production tools, ParaView and VisIt for the visualization of the simulation data.
This demonstration will provide a brief overview of a suite of HPC monitoring and analysis tools developed in collaboration between Sandia National Laboratories (SNL), Los Alamos National Laboratories (LANL), and Open Grid Computing (OGC). The demonstration will provide a highlight overview of: 1) our Lightweight Distributed Metric Service (LDMS) for data collection, transport, and storage (including use case examples of system and job based analyses with visualization) and 2) Baler tool for mapping log messages into patterns and performing a variety of pattern based analyses. For additional information about our suite of HPC monitoring and analysis tools please email ovis-help@sandia.gov or visit http://www.opengridcomputing.com/sc18
Wednesday, Nov. 14
Demo Station 1
In situ visualization and analysis is an important component of the path to exascale computing. Coupling simulation codes directly to analysis codes reduces their I/O while increasing the temporal fidelity of the analysis. SENSEI, a light weight in situ frame work, gives simulations access to a diverse set of analysis back ends through a simple API and data model. SENSEI currently supports ParaView Catalyst, VisIt Libsim, ADIOS, Python, and VTK-m based back ends and is easy to extend. In this presentation we introduce SENSEI and demonstrate its use with the IAMR an AMReX based compressible Navier Stokes simulation code.
As part of the ECP CODAR project, Brookhaven National Laboratory, in collaboration with the Oregon Universities TAU team, has developed unique capabilities to analyze, reduce and visualize single application and complete workflow performance data in-situ. The resulting tool enables the researchers to examine and explore their workflow performance as it is being executed.
As part of the ECP CODAR project, Brookhaven National Laboratory, in collaboration with the Oregon Universities TAU team, has developed unique capabilities to analyze, reduce and visualize single application and complete workflow performance data in-situ. The resulting tool enables the researchers to examine and explore their workflow performance as it is being executed.
Charliecloud provides user-defined software stacks (UDSS) for HPC centers. This “bring your own software stack” functionality addresses needs such as: software dependencies that are numerous, complex, unusual, differently configured, or simply newer/older than what the center provides; build-time requirements unavailable within the center, such as relatively unfettered internet access; validated software stacks and configuration to meet the standards of a particular field of inquiry; portability of environments between resources, including workstations and other test and development system not managed by the center; consistent environments, even archivally so, that can be easily, reliably, and verifiably reproduced in the future; and/or usability and comprehensibility. Charliecloud uses Linux user namespaces to run containers with no privileged operations or daemons and minimal configuration changes on center resources. This simple approach avoids most security risks while maintaining access to the performance and functionality already on offer. Container images can be built using Docker or anything else that can generate a standard Linux filesystem tree. We will present a brief introduction to Charliecloud, then demonstrate running portable Charliecloud containers of various flavors at native speed, including hello world, traditional MPI, data-intensive (e.g., Apache Spark), and GPU-accelerated (e.g., TensorFlow).
The superfacility vision combines multiple complementary user facilities into a virtual facility offering fundamentally greater capability than the standalone facilities provide on their own. For example, integrating beamlines at the Advanced Light Source (ALS), with HPC resources at NERSC via ESnet provides scientific capabilities unavailable at any single facility. This use of disparate facilities is not always convenient, and the logistics of setting up multiple user accounts, and managing multiple credentials adds unnecessary friction to the scientific process. We will demonstrate a simple portal, based on off-the-shelf technologies, that combines federated authentication with metadata collected at the time of the experiment and preserved at the HPC facility to allow a scientist to use their home institutional identity and login processes to access superfacility experimental data and results.
As the volume and velocity of data generated by experiments continues to increase, we find the need to move data analysis and reduction operations closer to the source of the data to reduce the burden on existing HPC facilities that threaten to be overrun by the surge of experimental and observational data. Furthermore, remote facilities, including astronomy observatories, particle accelerators such as SLAC LCLS-II, etc. producing data do not necessarily have dedicated HPC facilities on-site. These remote sites are often power or space constrained, making the construction of a traditional data center or HPC facility unreasonable. Further complicating these scenarios, each experiment often needs a blend of specialized and programmable hardware that is closely tied to the needs of the individual experiment. We propose a hardware generation methodology based on open-source components to rapidly design and deploy these data filtering and analysis computing devices. He re we demonstrate a potential near-sensor, real-time, data processing solution developed using an innovative open-source hardware generation technique allowing potentially more effective use of experimental devices, such as electron microscopy.
Demo Station 2
This demonstration will provide a brief overview of a suite of HPC monitoring and analysis tools developed in collaboration between Sandia National Laboratories (SNL), Los Alamos National Laboratories (LANL), and Open Grid Computing (OGC). The demonstration will provide a highlight overview of: 1) our Lightweight Distributed Metric Service (LDMS) for data collection, transport, and storage (including use case examples of system and job based analyses with visualization) and 2) Baler tool for mapping log messages into patterns and performing a variety of pattern based analyses. For additional information about our suite of HPC monitoring and analysis tools please email ovis-help@sandia.gov or visit http://www.opengridcomputing.com/sc18
This demonstration will provide a brief overview of a suite of HPC monitoring and analysis tools developed in collaboration between Sandia National Laboratories (SNL), Los Alamos National Laboratories (LANL), and Open Grid Computing (OGC). The demonstration will provide a highlight overview of: 1) our Lightweight Distributed Metric Service (LDMS) for data collection, transport, and storage (including use case examples of system and job based analyses with visualization) and 2) Baler tool for mapping log messages into patterns and performing a variety of pattern based analyses. For additional information about our suite of HPC monitoring and analysis tools please email ovis-help@sandia.gov or visit http://www.opengridcomputing.com/sc18
The ECP SDK project is providing software developed under the ECP project using Spack [http://www.spack.io] as the primary means of software distribution. Using Spack, we have also created container images of packaged ECP ST products in the Docker, Singularity, Shifter, and Charliecloud environments that may be deployed on HPC systems. This demo will show how to use these container images for software development and describe packaging software in Spack. These images will be distributed on USB sticks.
This demonstration presents Spin, a Docker-based platform at NERSC that enables researchers to design, build, and manage their own science gateways and other services to complement their computational jobs, present data or visualizations produced by computational processes, conduct complex workflows, and more. After explaining the rationale behind building Spin and describing its basic architecture, staff will show how services can be created in just a few minutes using simple tools. A discussion of services implemented with Spin and ideas for the future will follow.
ParaView will be running with large data on a remote cluster at Sandia National Laboratories.
Thursday, Nov. 15
Demo Station 1
This demo will present an overview and some key software components of the SOLLVE ECP project. SOLLVE aims at enhancing OpenMP to cover the major requirements of ECP application codes. In addition, this project sets the goal to deliver a high-quality, robust implementation of OpenMP and project extensions in LLVM, an open source compiler infrastructure with an active developer community that impacts the DOE pre-exascale systems (CORAL). This project further develops the LLVM BOLT runtime system to exploit light-weight threading for scalability and facilitate interoperability with MPI. SOLLVE is also creating a validation suite to assess our progress and that of vendors to ensure that quality implementations of OpenMP are being delivered to Exascale systems. The project also encourages the accelerated development of similarly high-quality, complete vendor implementations and facilitate extensive interactions between the applications developers and OpenMP developers in industry.